Semi-supervised retinal OCT image layer segmentation method combined with generative adversarial network
A retinal and semi-supervised technology, applied in image analysis, biological neural network models, image enhancement, etc., can solve the problems of low segmentation accuracy, lack of training data, low model generalization ability, etc., and achieve enhanced robustness Effect
- Summary
- Abstract
- Description
- Claims
- Application Information
AI Technical Summary
Problems solved by technology
Method used
Image
Examples
Embodiment
[0030] The present invention proposes a semi-supervised retinal OCT image layer (also called retinal OCT image semantics) segmentation algorithm through confrontational learning. A typical GAN consists of two sub-networks, the generator and the discriminator, which play against each other during training. In the present invention, a segmentation network and a discriminator network are introduced, both of which are fully convolutional networks, and the accuracy of segmentation is improved by introducing unlabeled training pictures.
[0031] Such as Figure 1-4 Shown, the present invention comprises the steps:
[0032] 1. Retina OCT image data preparation
[0033] This method was used in the publicly available SD-OCT dataset of DME patients by Chiu et al. , 6(4):1172.) for evaluation. The dataset contains 10 patients, and each patient contains 61 SD-OCT images; of the 61 images per patient, 11 images were annotated by two expert clinicians as retinal layers and fluid regio...
PUM
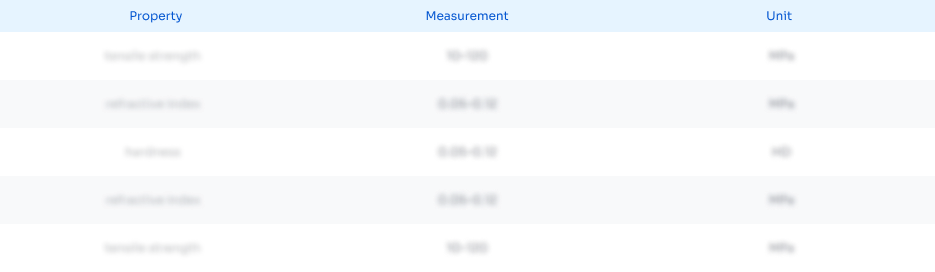
Abstract
Description
Claims
Application Information
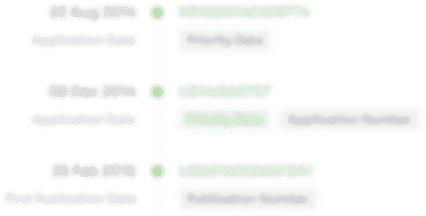
- R&D Engineer
- R&D Manager
- IP Professional
- Industry Leading Data Capabilities
- Powerful AI technology
- Patent DNA Extraction
Browse by: Latest US Patents, China's latest patents, Technical Efficacy Thesaurus, Application Domain, Technology Topic, Popular Technical Reports.
© 2024 PatSnap. All rights reserved.Legal|Privacy policy|Modern Slavery Act Transparency Statement|Sitemap|About US| Contact US: help@patsnap.com