Underwater acoustic target recognition method based on deep convolutional generative adversarial network
A technology of target recognition and deep convolution, applied in biological neural network models, neural learning methods, character and pattern recognition, etc., can solve problems such as relying on manual experience, cumbersome feature extraction procedures, and difficult to fully and effectively use
- Summary
- Abstract
- Description
- Claims
- Application Information
AI Technical Summary
Problems solved by technology
Method used
Image
Examples
Embodiment Construction
[0024] Combine below figure 1 The present invention is further described.
[0025] Using python as the operating platform, the detailed implementation steps and calculation methods of this method are listed.
[0026] Introduction to the database of this example: The lake test data set is divided into 4 categories, which are the radiation noise of 4 different types of ships. The types of ships are iron boat, Shuhang, Cathay and New Century. Array for round-the-clock data collection. In this experiment, the data of one of the channels of one of the linear arrays is intercepted, and the data of each type of target is intercepted for 20 minutes, and the sampling frequency is 48kHz.
[0027] Such as figure 1 Shown:
[0028] Step 1: Normalize the original underwater acoustic signal, divide into frames with a frame length of 0.01s and a frame overlap of 0.005s, and obtain 240,000 samples of each category, a total of 960,000 samples. Among them, 40,000 samples are randomly select...
PUM
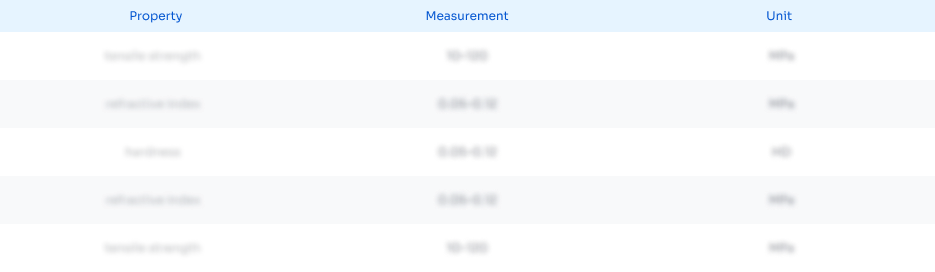
Abstract
Description
Claims
Application Information
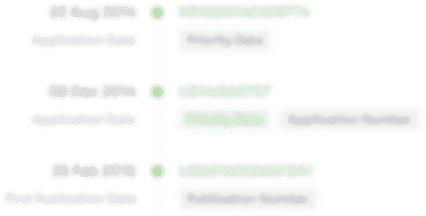
- R&D Engineer
- R&D Manager
- IP Professional
- Industry Leading Data Capabilities
- Powerful AI technology
- Patent DNA Extraction
Browse by: Latest US Patents, China's latest patents, Technical Efficacy Thesaurus, Application Domain, Technology Topic, Popular Technical Reports.
© 2024 PatSnap. All rights reserved.Legal|Privacy policy|Modern Slavery Act Transparency Statement|Sitemap|About US| Contact US: help@patsnap.com