Hip joint X-ray image segmentation method and system based on local vision clue
A technology of visual cues and light images, applied in the field of deep learning and computer vision, can solve limited problems, achieve the effect of reducing complexity, model robustness, and improving model effect
- Summary
- Abstract
- Description
- Claims
- Application Information
AI Technical Summary
Problems solved by technology
Method used
Image
Examples
Embodiment Construction
[0028] The present invention will be further described below in conjunction with the accompanying drawings.
[0029] figure 1 It is a schematic flowchart of a method for segmenting hip joint X-ray images based on local visual cues provided by an embodiment of the present invention, and the method includes the following steps:
[0030] Step 101, obtaining a dataset marked with X-ray images, preprocessing the image data in the dataset, obtaining a hip joint region (ROI) based on segmentation and labeling, and normalizing the ROI region;
[0031] Specifically, when preprocessing the image data in the data set, you can first use the labelme labeling tool to obtain the X-ray image labeling data set, convert the data set format to COCO2014 format, and use the pycocotools toolkit to deserialize the json to obtain the network Input, recalculate the ROI area according to the range of the segmented points and the random number, enhance the data, and normalize to 128x128.
[0032] Step...
PUM
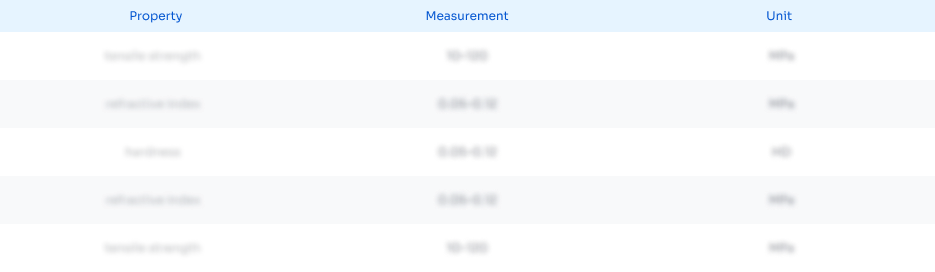
Abstract
Description
Claims
Application Information
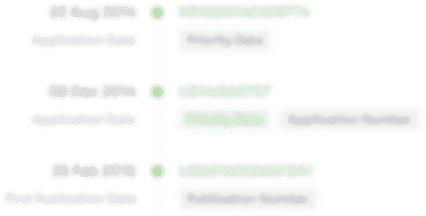
- R&D Engineer
- R&D Manager
- IP Professional
- Industry Leading Data Capabilities
- Powerful AI technology
- Patent DNA Extraction
Browse by: Latest US Patents, China's latest patents, Technical Efficacy Thesaurus, Application Domain, Technology Topic, Popular Technical Reports.
© 2024 PatSnap. All rights reserved.Legal|Privacy policy|Modern Slavery Act Transparency Statement|Sitemap|About US| Contact US: help@patsnap.com