Method for improving quality of deep learning data set and interpretability of model based on mesoscience guidance
A deep learning and data set technology, applied in the field of artificial intelligence, can solve problems such as poor model interpretability, and achieve the effect of improving representativeness and effectiveness, reducing the amount of data, and good interpretability
- Summary
- Abstract
- Description
- Claims
- Application Information
AI Technical Summary
Problems solved by technology
Method used
Image
Examples
Embodiment 1
[0019] Example 1: Recognition of the watershed and its average void ratio of a 3D gas-solid flow system based on a 2D simulation image
[0020] First, the unit of the gas-solid flow system is determined to be a single solid particle, and the problem to be dealt with only includes one system, and the mesoscale is the particle aggregate scale. There are two types of extreme mesoscale structures in this system: one is that the size of particle agglomerates is as large as the system size, that is, a fixed bed, and the corresponding dominant mechanism is that the average void ratio tends to the minimum value; the other is that the size of particle agglomerates is as small as The cell size, ie dilute phase transport, corresponds to the dominant mechanism where the rate of energy expenditure for suspending and transporting particles within a unit volume of the bed tends towards a minimum. The intermediate area between fixed bed and dilute phase transport is fluidized bed, whose stabi...
Embodiment 2
[0021] Example 2: Recognition of the watershed and its average void ratio of the 3D gas-solid flow system based on the 2D experimental image
[0022]First, the unit of the gas-solid flow system is determined to be a single solid particle, and the problem to be dealt with only includes one system, and the mesoscale is the particle aggregate scale. There are two types of extreme mesoscale structures in this system: one is that the size of particle agglomerates is as large as the system size, that is, a fixed bed, and the corresponding dominant mechanism is that the average void ratio tends to the minimum value; the other is that the size of particle agglomerates is as small as The cell size, ie dilute phase transport, corresponds to the dominant mechanism where the rate of energy expenditure for suspending and transporting particles within a unit volume of the bed tends towards a minimum. The intermediate area between fixed bed and dilute phase transport is fluidized bed, whose ...
PUM
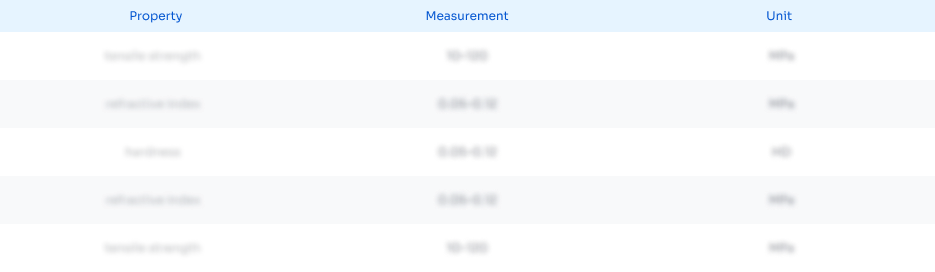
Abstract
Description
Claims
Application Information
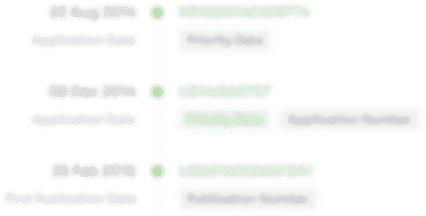
- R&D
- Intellectual Property
- Life Sciences
- Materials
- Tech Scout
- Unparalleled Data Quality
- Higher Quality Content
- 60% Fewer Hallucinations
Browse by: Latest US Patents, China's latest patents, Technical Efficacy Thesaurus, Application Domain, Technology Topic, Popular Technical Reports.
© 2025 PatSnap. All rights reserved.Legal|Privacy policy|Modern Slavery Act Transparency Statement|Sitemap|About US| Contact US: help@patsnap.com