FPGA (Field Programmable Gate Array)-based YOLOv2-tiny neural network low-delay hardware accelerator implementation method
A hardware accelerator and neural network technology, applied in biological neural network models, physical implementation, neural architecture, etc., can solve the problem of high delay of hardware accelerators, achieve the effects of reducing startup time, improving usage efficiency, and reducing computing time
- Summary
- Abstract
- Description
- Claims
- Application Information
AI Technical Summary
Problems solved by technology
Method used
Image
Examples
Embodiment Construction
[0053] In order to make the measures, creative features, goals and effects achieved by the present invention easy to understand, the present invention will be further described below in conjunction with the accompanying drawings and embodiments.
[0054] The present invention is an FPGA-based YOLOv2-tiny neural network low-latency hardware accelerator implementation method, the hardware platform used is Xilinx ZC706 development board, the data set selected for training and testing is Kitti, and the input picture size is 1280×384, specifically The network structure is shown in Table 1.
[0055] Table 1 YOLOv2-tiny network structure
[0056] name The main parameters input size output size Conv1 Convolution layer, convolution kernel (3,3,16) (1280,384,3) (1280,384,16) BN1 batch normalization layer (1280,384,16) (1280,384,16) Maxpool1 pooling layer, pooling kernel(2,2) (1280,384,16) (640,192,16) Conv2 Convolution layer, convolution...
PUM
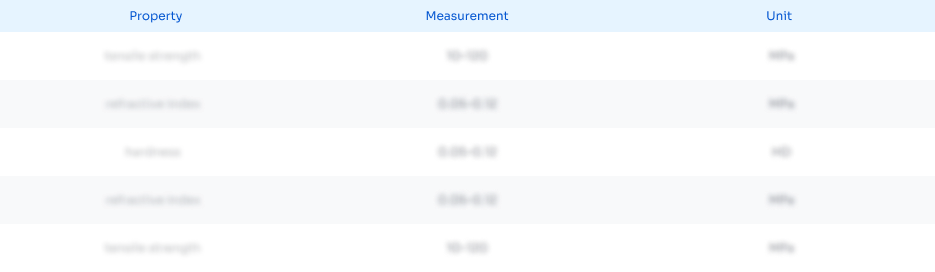
Abstract
Description
Claims
Application Information
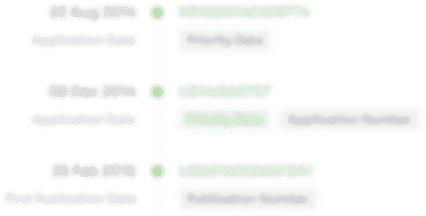
- R&D Engineer
- R&D Manager
- IP Professional
- Industry Leading Data Capabilities
- Powerful AI technology
- Patent DNA Extraction
Browse by: Latest US Patents, China's latest patents, Technical Efficacy Thesaurus, Application Domain, Technology Topic, Popular Technical Reports.
© 2024 PatSnap. All rights reserved.Legal|Privacy policy|Modern Slavery Act Transparency Statement|Sitemap|About US| Contact US: help@patsnap.com