Model construction method based on transfer learning, image recognition method and device
A technology for learning models and building methods, applied in the field of image processing, which can solve problems such as limiting the application scope of learning models, poor object recognition experience, and object recognition that cannot distinguish features.
- Summary
- Abstract
- Description
- Claims
- Application Information
AI Technical Summary
Problems solved by technology
Method used
Image
Examples
Embodiment 1
[0058] Please refer to figure 1 , the present application provides a method for building a model based on transfer learning, including steps S100-S300, which are described below.
[0059] Step S100, using the first training set to obtain a first learning model through deep neural network training.
[0060] It should be noted that the deep neural network here can be a long-term short-term memory network (Long / short termmemory, referred to as LSTM), a recurrent neural network (Recurrent neural networks, referred to as RNN), a generative adversarial network (Generative adversarial networks, referred to as GAN), Convolutional neural networks (DCNN for short) or deep convolutional inverse graphics networks (DCIGN for short), are not limited here.
[0061] It should be noted that the first training set here includes multiple images of objects with the first type of features, for example, the first training set includes multiple face images of Asians, where the first type of feature...
Embodiment 2
[0113] Please refer to Figure 5 , on the basis of the model construction method disclosed in Embodiment 1, the present application also discloses an image recognition method, which includes steps S410-S430, which will be described separately below.
[0114] Step S410, acquiring an image of an object to be detected, where the object to be detected is an object having a first type of feature and / or a second type of feature.
[0115] For example, if the object to be detected is an Asian, the first type of features corresponding to the Asian may include feature information on yellow skin, black eyes, black hair, and diamond-shaped facial contours. If the object to be detected is a European, then the second type of features corresponding to the European may include feature information of white skin color, blue eyes, blond hair, square face outline, and the like. If the object to be detected is a Eurasian mixed race, then his (her) face may include several feature information in t...
Embodiment 3
[0123] Please refer to Figure 6, on the basis of the image recognition method disclosed in Embodiment 2, correspondingly, the present application also discloses an image recognition device 1, which mainly includes an image acquisition unit 11, a feature extraction unit 12 and an object recognition unit 13, which will be described separately below .
[0124] The image acquiring unit 11 is configured to acquire an image of an object to be detected, and the object to be detected is an object having a first type of feature and / or a second type of feature. Specifically, the image acquisition unit 11 can acquire images of the object to be detected by means of imaging devices such as cameras and cameras, or even media videos. Regarding the specific functions of the image acquisition unit 11 , reference may be made to step S410 in the second embodiment, which will not be repeated here.
[0125] The feature extraction unit 12 is used to extract feature information in the image of th...
PUM
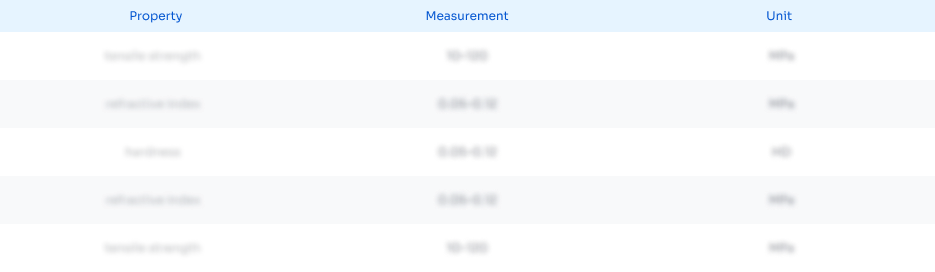
Abstract
Description
Claims
Application Information
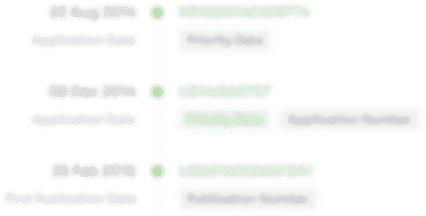
- R&D Engineer
- R&D Manager
- IP Professional
- Industry Leading Data Capabilities
- Powerful AI technology
- Patent DNA Extraction
Browse by: Latest US Patents, China's latest patents, Technical Efficacy Thesaurus, Application Domain, Technology Topic, Popular Technical Reports.
© 2024 PatSnap. All rights reserved.Legal|Privacy policy|Modern Slavery Act Transparency Statement|Sitemap|About US| Contact US: help@patsnap.com