Transformer DGA data prediction method based on multi-dimensional time sequence frame convolution LSTM
A data prediction and transformer technology, applied in the field of power transformer fault prediction, can solve problems such as difficult to deal with data correlation, massive data, etc., and achieve the effect of accurate prediction
- Summary
- Abstract
- Description
- Claims
- Application Information
AI Technical Summary
Problems solved by technology
Method used
Image
Examples
Embodiment Construction
[0039] In order to make the object, technical solution and advantages of the present invention more clear, the present invention will be further described in detail below in conjunction with the accompanying drawings and embodiments. It should be understood that the specific embodiments described here are only used to explain the present invention, not to limit the present invention.
[0040] The invention is not only applicable to the prediction method of the dissolved gas components in the transformer oil, but also can be extended to other prediction fields.
[0041] The present invention comprehensively considers the complex relationship between the dissolved gas components in various transformer oils, between the front and rear time series, and between different devices, constructs the time series frame and performs feature extraction through the convolution layer, and finally uses the LSTM network to realize the dissolution in the oil Failure prediction of gas components....
PUM
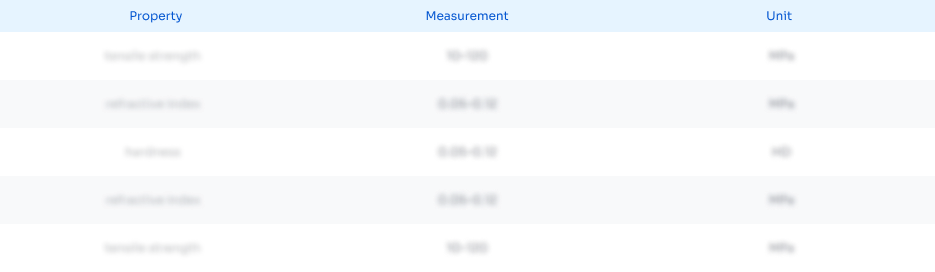
Abstract
Description
Claims
Application Information
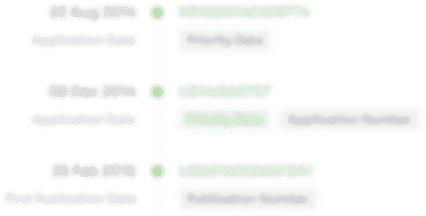
- R&D
- Intellectual Property
- Life Sciences
- Materials
- Tech Scout
- Unparalleled Data Quality
- Higher Quality Content
- 60% Fewer Hallucinations
Browse by: Latest US Patents, China's latest patents, Technical Efficacy Thesaurus, Application Domain, Technology Topic, Popular Technical Reports.
© 2025 PatSnap. All rights reserved.Legal|Privacy policy|Modern Slavery Act Transparency Statement|Sitemap|About US| Contact US: help@patsnap.com