Integrated learning based mountain wind generation set behavior predicating model
A technology for wind turbines and prediction models, applied in wind power generation, wind turbines, engines, etc., can solve the problem that the mechanism model does not consider the operating environment of the wind turbine in the mountains, affects the output of the wind turbine model, and affects the prediction accuracy of the model. generalization ability, superior generalization ability, and the effect of preventing overfitting
- Summary
- Abstract
- Description
- Claims
- Application Information
AI Technical Summary
Problems solved by technology
Method used
Image
Examples
Embodiment Construction
[0018] The mountain wind turbine modeling method based on integrated learning proposed by the present invention includes steps:
[0019] Step 1: Collect wind turbine data for one year from the SCADA system of the wind farm, and the sampling interval is Second. data can be expressed as , respectively Wind speed, wind direction, 5-second yaw-to-wind average, fan output power, pitch angle, fan rotor speed, blade acceleration, and blade angle at all times. The collected data is cleaned by the density method for abnormal data, where the density threshold Determined by adaptive thresholding. The specific steps are as follows:
[0020] Step 1-1: Wind Speed ,wind direction , 5 seconds yaw and wind average , fan output power , pitch angle , fan rotor speed , blade acceleration , blade angle and time t Respectively as the columns of the matrix, form the matrix ,Right now
[0021] (1)
[0022] Step 1-2: According to the principle of increasing wind spee...
PUM
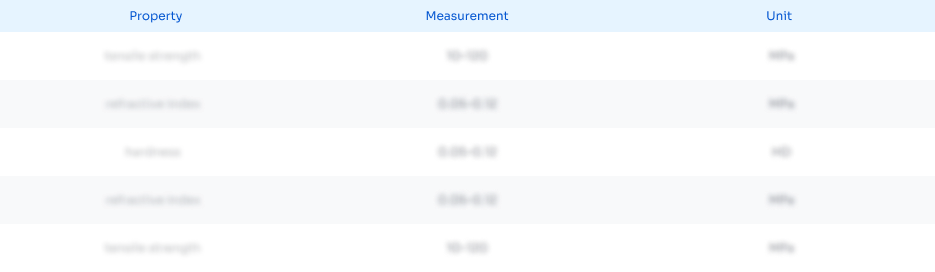
Abstract
Description
Claims
Application Information
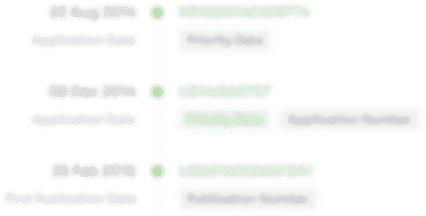
- R&D Engineer
- R&D Manager
- IP Professional
- Industry Leading Data Capabilities
- Powerful AI technology
- Patent DNA Extraction
Browse by: Latest US Patents, China's latest patents, Technical Efficacy Thesaurus, Application Domain, Technology Topic.
© 2024 PatSnap. All rights reserved.Legal|Privacy policy|Modern Slavery Act Transparency Statement|Sitemap