Based on CNN semantic segmentation depth prediction method and device
A technology of depth prediction and semantic segmentation, applied in neural learning methods, image analysis, image enhancement, etc., can solve problems such as inability to solve depth problems, insufficient robustness of geometric consistency, etc., and achieve the effect of optimizing the inaccurate absolute scale
- Summary
- Abstract
- Description
- Claims
- Application Information
AI Technical Summary
Problems solved by technology
Method used
Image
Examples
Embodiment Construction
[0045] The experimental data set used in this application is the KITTI data set (co-founded by Karlsruhe Institute of Technology in Germany and Toyota American Institute of Technology), which is currently the largest computer vision algorithm evaluation data set in the world for autonomous driving scenarios. The acquisition platform of the KITTI dataset includes: 2 grayscale cameras, 2 color cameras, a Velodyne 3D lidar, 4 optical lenses, and a GPS navigation system. The entire dataset consists of 389 pairs of stereo images and optical flow maps, 39.2 km visual odometry sequences, and more than 200,000 images of 3D annotated objects, each of which includes up to 15 vehicles and 30 pedestrians, and also contains varying degrees of occlude.
[0046] figure 1 This is a flowchart of a method for depth prediction based on CNN semantic segmentation according to an embodiment of the present application. see figure 1 , the method includes:
[0047] 101: Obtain the current frame fr...
PUM
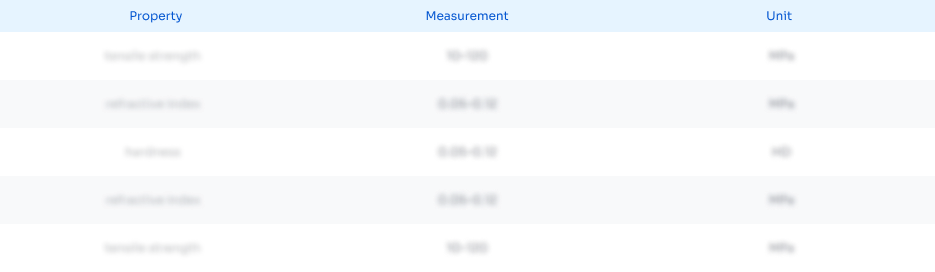
Abstract
Description
Claims
Application Information
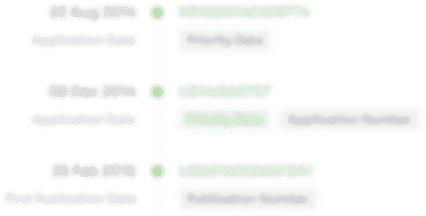
- R&D Engineer
- R&D Manager
- IP Professional
- Industry Leading Data Capabilities
- Powerful AI technology
- Patent DNA Extraction
Browse by: Latest US Patents, China's latest patents, Technical Efficacy Thesaurus, Application Domain, Technology Topic, Popular Technical Reports.
© 2024 PatSnap. All rights reserved.Legal|Privacy policy|Modern Slavery Act Transparency Statement|Sitemap|About US| Contact US: help@patsnap.com