Composite fault diagnosis method for rotary machine based on improved deep sparse auto-encoder network
A technology of sparse autoencoder and rotating machinery, which is applied in neural learning methods, biological neural network models, and testing of machine/structural components. Reduce the self-adaptability of diagnostic methods and other issues
- Summary
- Abstract
- Description
- Claims
- Application Information
AI Technical Summary
Problems solved by technology
Method used
Image
Examples
Embodiment Construction
[0075] The specific implementation of the present invention will be further described in detail below in conjunction with the accompanying drawings, attached tables and examples.
[0076] In order to make up for the deficiencies in the prior art, the present invention is based on the relationship constraint item and the improved deep sparse autoencoder network rotating machinery compound fault diagnosis method, firstly establishes the relationship constraint item that alleviates the correlation between data, and adopts the improved deep sparse autoencoder network The self-encoder network is used to learn the essential characteristics of the training sample data; then the softmax classifier is used to classify and identify the test samples, so as to determine the category of the composite fault condition of the rotating machinery and the severity of the fault, so as to improve the accuracy of the composite fault diagnosis of the rotating machinery , Adaptability, Effectiveness a...
PUM
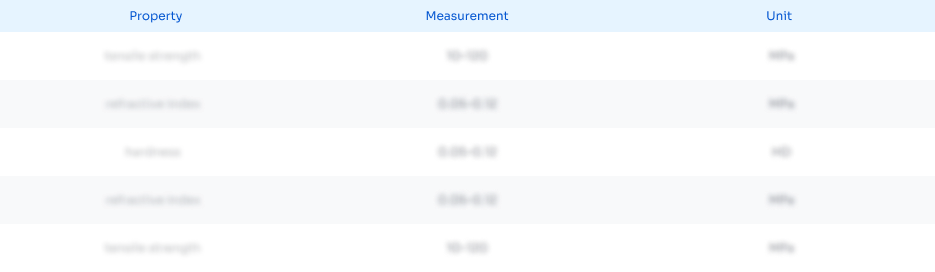
Abstract
Description
Claims
Application Information
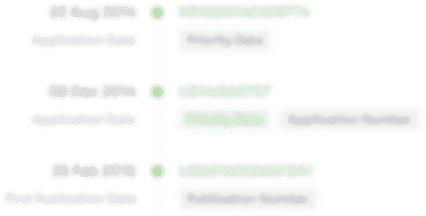
- R&D Engineer
- R&D Manager
- IP Professional
- Industry Leading Data Capabilities
- Powerful AI technology
- Patent DNA Extraction
Browse by: Latest US Patents, China's latest patents, Technical Efficacy Thesaurus, Application Domain, Technology Topic, Popular Technical Reports.
© 2024 PatSnap. All rights reserved.Legal|Privacy policy|Modern Slavery Act Transparency Statement|Sitemap|About US| Contact US: help@patsnap.com