Nonlinear industrial process robust identification and output estimation method
It is an industrial process and non-linear technology, which is applied in computing, manufacturing computing systems, complex mathematical operations, etc., and can solve problems such as the reduction of system identification accuracy.
- Summary
- Abstract
- Description
- Claims
- Application Information
AI Technical Summary
Problems solved by technology
Method used
Image
Examples
specific Embodiment approach 1
[0081] Specific implementation mode one: refer to figure 1 Specifically explaining this embodiment, a nonlinear industrial process robust identification and output estimation method described in this embodiment includes the following steps:
[0082] Step 1: Select the local model of the system, and establish a robust probability model of the multi-model nonlinear system based on the Laplace distribution;
[0083] Step 2: According to the variational Bayesian framework, establish the posterior distribution of hidden variables and the iterative update formula of the parameters to be estimated;
[0084] Step 3: Set the posterior distribution of hidden variables established in step 2 and the termination condition of the iterative update formula of the parameters to be estimated. When the iteration is terminated, record the final iteration result as the estimated optimal parameter, and then obtain it through local model interpolation Model output value.
[0085] Detailed steps of...
Embodiment
[0149] (1) Select a first-order process whose transfer function is as follows:
[0150]
[0151] where K(w)=w 2 +0.6 is the system gain, τ(w)=0.5w 3 +3 is the system time constant, and the value range of scheduling variable w is w∈[1,4]. Because the system gain and time constant can vary by more than 10 times in this working range, it is difficult for a single linear model to describe the dynamic characteristics of the system. The identification experiment of this nonlinear process is now carried out by means of weighted combination of multiple local ARX models. Three operating points are chosen: w=1, w=2.25 and w=4. The scheduling variables vary as follows:
[0152] 1~100s: at the working point w=1;
[0153] 101~400s: change linearly from working point w=1 to working point w=2.25;
[0154] 401~550s: at the working point w=2.25;
[0155] 551~750s: linear change from working point w=1 to working point w=4;
[0156] 751~900s: at the working point w=...
PUM
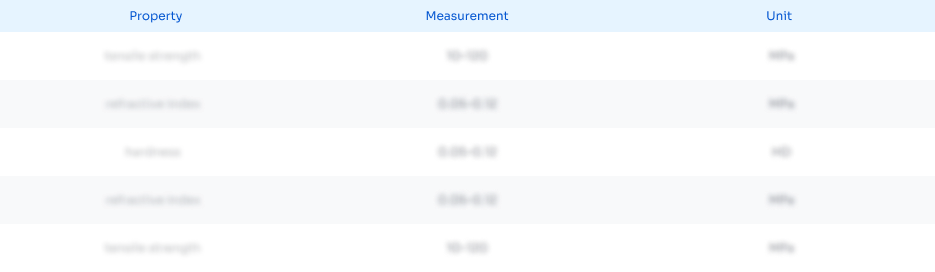
Abstract
Description
Claims
Application Information
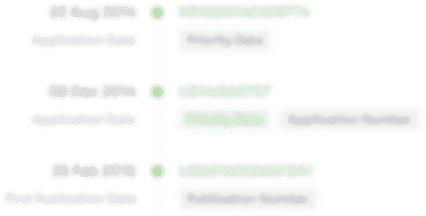
- R&D Engineer
- R&D Manager
- IP Professional
- Industry Leading Data Capabilities
- Powerful AI technology
- Patent DNA Extraction
Browse by: Latest US Patents, China's latest patents, Technical Efficacy Thesaurus, Application Domain, Technology Topic, Popular Technical Reports.
© 2024 PatSnap. All rights reserved.Legal|Privacy policy|Modern Slavery Act Transparency Statement|Sitemap|About US| Contact US: help@patsnap.com