Robust control method based on reinforcement learning and Lyapunov function
A technology of reinforcement learning and robust control, applied in the direction of adaptive control, general control system, control/regulation system, etc., can solve the problems of low safety and stability, to ensure free exploration, high efficiency and safety Effect
- Summary
- Abstract
- Description
- Claims
- Application Information
AI Technical Summary
Problems solved by technology
Method used
Image
Examples
Embodiment 1
[0038] like figure 1 Shown is an embodiment of a robust control method based on reinforcement learning and Lyapunov functions, including the following steps:
[0039] Step 1: Build an affine system model, and then model the uncertainty in the system dynamics model based on the Gaussian process; the nonlinear affine system can be modeled as: Among them, f(s)+g(s)a represents the prior model obtained by modeling system dynamics and kinematics, and d(s) represents the deviation between the model and the real environment; Gaussian process regression obtains the state s through Bayesian inference * The lower deviation d(s * ) mean and variance:
[0040]
[0041]
[0042] Among them, k(s i ,s j ) is the kernel function defined in GP, k n =[k(s 1 ,s * ), k(s 2 ,s * ),...,k(s n ,s * )], [K] i,j =k(s i ,s j ) is the kernel matrix, is the label vector, σ noise is the standard deviation of label data noise, I is the identity matrix;
[0043] Get a high confiden...
Embodiment 2
[0060] like Figure 1-3 As shown, on the basis of Embodiment 1, this embodiment takes the trajectory tracking and obstacle avoidance tasks of a quadrotor UAV under random wind field interference as an example to illustrate the specific implementation steps of this method:
[0061] Step 1: In this example, based on the prior knowledge of robot dynamics and the actual task scene, a baseline nonlinear affine system model is established, and a parameterized trajectory in three-dimensional space is set, including the trajectory start point and end point, which is recorded as r(t) ∈ R 3 .
[0062] Define the safe state space of the UAV according to the task scenario: C={x|h(x)≥0} and the target balance point, such as: avoiding obstacles, the trajectory point as the balance point, and designing the corresponding control barrier function (CBF )h(s t ) and the Lyapunov function (CLF) V(s t ).
[0063] Step 2: Select the framework of model predictive control as the benchmark strate...
PUM
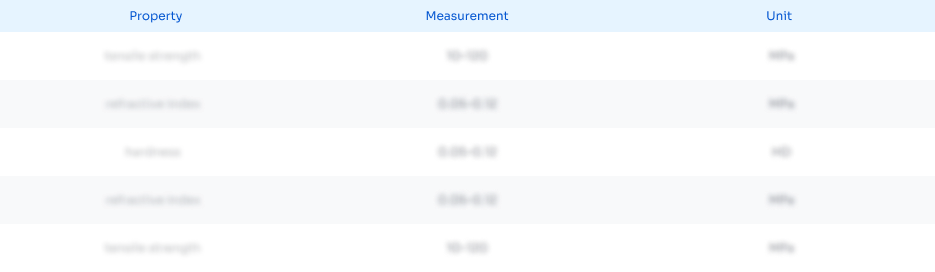
Abstract
Description
Claims
Application Information
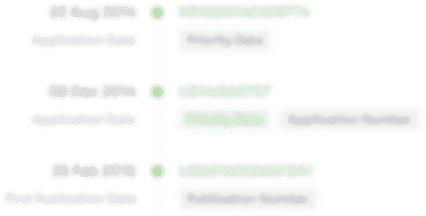
- R&D Engineer
- R&D Manager
- IP Professional
- Industry Leading Data Capabilities
- Powerful AI technology
- Patent DNA Extraction
Browse by: Latest US Patents, China's latest patents, Technical Efficacy Thesaurus, Application Domain, Technology Topic, Popular Technical Reports.
© 2024 PatSnap. All rights reserved.Legal|Privacy policy|Modern Slavery Act Transparency Statement|Sitemap|About US| Contact US: help@patsnap.com