Multi-task learning method for real-time target detection and semantic segmentation based on lightweight network
A multi-task learning and semantic segmentation technology, which is applied in the direction of instruments, character and pattern recognition, scene recognition, etc., can solve the problem of excessive size difference of objects, and achieve the effect of saving model size
- Summary
- Abstract
- Description
- Claims
- Application Information
AI Technical Summary
Problems solved by technology
Method used
Image
Examples
Embodiment 1
[0037] When implementing a multi-task learning method based on real-time target detection and semantic segmentation, it is first necessary to prepare training data, verification data, and test data, then perform model training and testing, and finally deploy it on unmanned vehicles.
[0038] 1) Preparation and processing of training data, verification data, and test data;
[0039] Step 1. Divide the BDD100K data set according to the ratio of 8:1:1 to obtain the corresponding training set, verification set and test set;
[0040] Step 2. Statistically measure the scale of each image detection object in the training set to facilitate subsequent verification;
[0041] Step 3. Perform data enhancement, image flipping, image cropping, brightness saturation change, and normalization processing on the training data to make full use of the data.
[0042] 2) Detailed process of model training:
[0043] Step 11. Use pytorch as the deep learning framework, pre-train MobileNet on ImageNe...
PUM
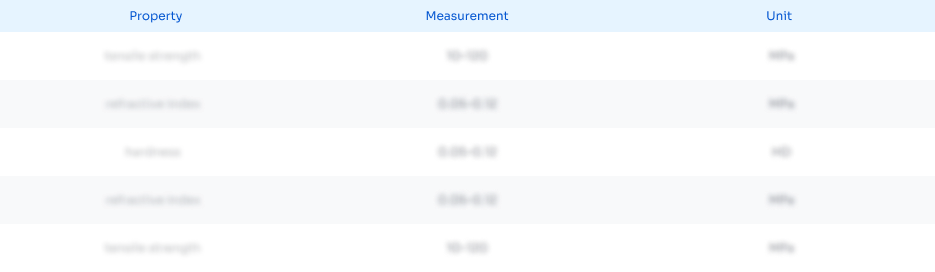
Abstract
Description
Claims
Application Information
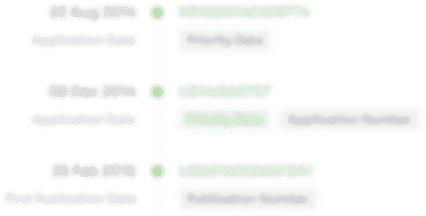
- R&D Engineer
- R&D Manager
- IP Professional
- Industry Leading Data Capabilities
- Powerful AI technology
- Patent DNA Extraction
Browse by: Latest US Patents, China's latest patents, Technical Efficacy Thesaurus, Application Domain, Technology Topic, Popular Technical Reports.
© 2024 PatSnap. All rights reserved.Legal|Privacy policy|Modern Slavery Act Transparency Statement|Sitemap|About US| Contact US: help@patsnap.com