Time sequence classification and retrieval method based on deep multi-task representation learning
A time-series, multi-task technology, applied in digital data information retrieval, character and pattern recognition, special data processing applications, etc., can solve the problem of not being able to capture unique information, and achieve the effect of avoiding information loss and improving accuracy
- Summary
- Abstract
- Description
- Claims
- Application Information
AI Technical Summary
Problems solved by technology
Method used
Image
Examples
Embodiment Construction
[0023] In order to make the object, technical solution and advantages of the present invention clearer, the present invention will be further described in detail below in conjunction with the accompanying drawings and embodiments. It should be understood that the specific embodiments described here are only used to explain the present invention, and do not limit the protection scope of the present invention.
[0024] The time series classification and retrieval method based on deep multi-task representation learning provided by the embodiment includes two stages: the construction of the time series classification model and the time series retrieval model and the application of the time series classification model and the time series retrieval model. The following is for each stage Describe in detail.
[0025] Construction phase of time series classification model and time series retrieval model
[0026] This stage mainly includes the construction of the training set, the cons...
PUM
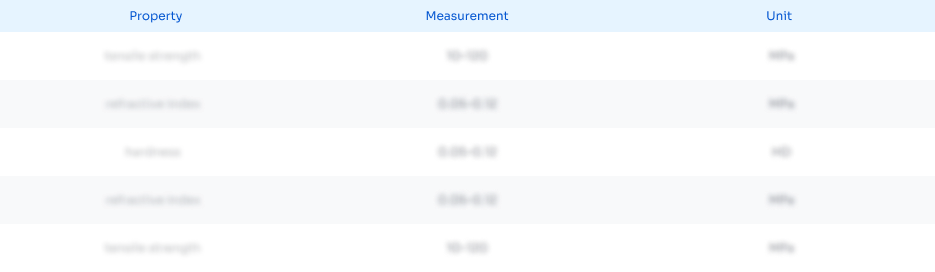
Abstract
Description
Claims
Application Information
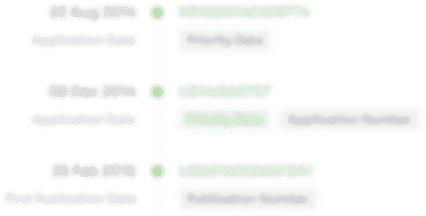
- R&D Engineer
- R&D Manager
- IP Professional
- Industry Leading Data Capabilities
- Powerful AI technology
- Patent DNA Extraction
Browse by: Latest US Patents, China's latest patents, Technical Efficacy Thesaurus, Application Domain, Technology Topic, Popular Technical Reports.
© 2024 PatSnap. All rights reserved.Legal|Privacy policy|Modern Slavery Act Transparency Statement|Sitemap|About US| Contact US: help@patsnap.com