Sample extraction method and image classification method based on hole convolution and residual link
An extraction method and sample technology, applied in the field of image processing, can solve the problems of poor self-adaptability and inability to obtain sufficient robust training samples, etc., and achieve the effect of strong self-adaptability and cognitive ability
- Summary
- Abstract
- Description
- Claims
- Application Information
AI Technical Summary
Problems solved by technology
Method used
Image
Examples
Embodiment Construction
[0035] In the process of biological vision forming scene recognition, only the central fovea of the human retina contains a large number of rod cells that can be clearly imaged, while the peripheral area can only be fuzzy imaged by cone cells, resulting in the need for micro-fibrillation (microsaccade, Also known as "micro-saccade", "micro-saccade"), the neural mechanism is to scan the scene by unconsciously and quickly turning the eyeballs to form an image of the entire scene. And in the process of microfibrillation, the blurred vision in the peripheral area of the retina provides probabilistic regional cognition, forming a priori reference, and on this basis, combined with the clear vision of the fovea, a complete and clear scene cognition is formed.
[0036] A sample extraction method, comprising the steps of:
[0037] Step 1: Get the original image Oimg, shrink Oimg n times, where, L represents the length of Oimg, W represents the width of Oimg, obtains an image pyra...
PUM
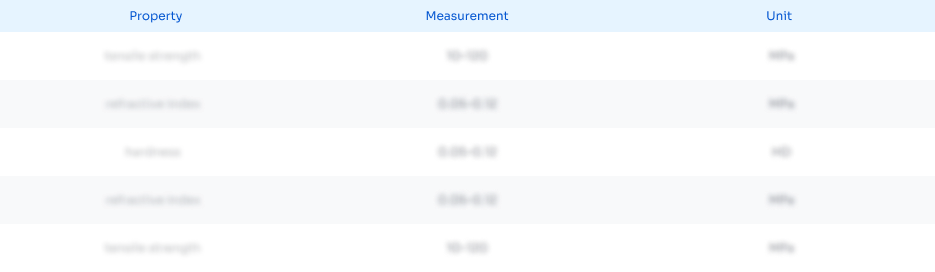
Abstract
Description
Claims
Application Information
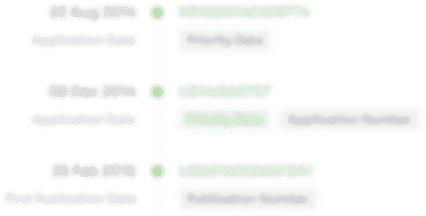
- R&D
- Intellectual Property
- Life Sciences
- Materials
- Tech Scout
- Unparalleled Data Quality
- Higher Quality Content
- 60% Fewer Hallucinations
Browse by: Latest US Patents, China's latest patents, Technical Efficacy Thesaurus, Application Domain, Technology Topic, Popular Technical Reports.
© 2025 PatSnap. All rights reserved.Legal|Privacy policy|Modern Slavery Act Transparency Statement|Sitemap|About US| Contact US: help@patsnap.com