Battery piece EL defect detection method based on improved SSD algorithm
A technology of defect detection and battery chip, which is applied in the field of defect detection and machine vision, can solve the problems of failing to meet the requirements of EL intelligent detection, the inability to meet the accuracy and efficiency, and the low precision of small-scale defects, so as to realize intelligent detection and improve Detection accuracy and speed, and the effect of improving detection performance
- Summary
- Abstract
- Description
- Claims
- Application Information
AI Technical Summary
Problems solved by technology
Method used
Image
Examples
Embodiment Construction
[0034] Embodiments of the present invention will be described in detail below in conjunction with the accompanying drawings.
[0035] An EL defect detection method based on SSD algorithm, including data set expansion, image labeling and training and testing of improved SSD network; specifically includes the following steps:
[0036] Step S1, EL image collection: collect EL defect images through a CCD camera;
[0037] Step S2, image data expansion: using rotation, the original image is horizontally rotated to expand the data set, and the final image is 1740, with a total of four types of defects;
[0038] Step S3, image labeling: using the labelImg tool to manually label images. During the labeling process, 11,450 objects were labeled from 1,740 images. The test set is randomly selected from the annotated images and contains 30% of the defects. Except for the test set, the remaining images are used as the training set;
[0039] Step S4, training and testing the improved SSD...
PUM
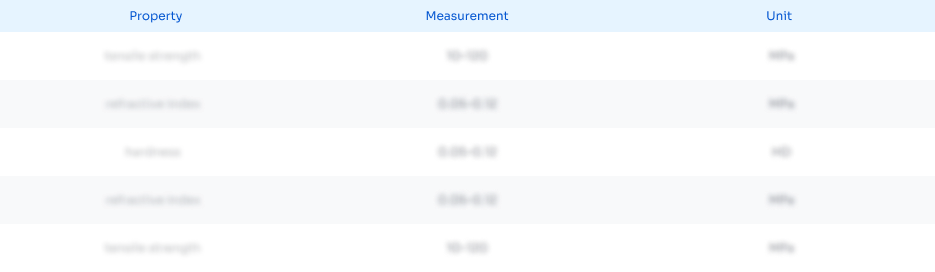
Abstract
Description
Claims
Application Information
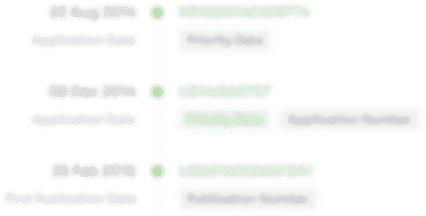
- R&D Engineer
- R&D Manager
- IP Professional
- Industry Leading Data Capabilities
- Powerful AI technology
- Patent DNA Extraction
Browse by: Latest US Patents, China's latest patents, Technical Efficacy Thesaurus, Application Domain, Technology Topic, Popular Technical Reports.
© 2024 PatSnap. All rights reserved.Legal|Privacy policy|Modern Slavery Act Transparency Statement|Sitemap|About US| Contact US: help@patsnap.com