Numerical mode correction method based on sequential regression learning
A numerical model and data technology, applied in the field of machine learning and weather forecasting, can solve problems such as limited accuracy of results, large amounts of data, and inability to generate forecast results, and achieve good correction results
- Summary
- Abstract
- Description
- Claims
- Application Information
AI Technical Summary
Problems solved by technology
Method used
Image
Examples
experiment example 1
[0068] Experimental Example 1: Performance of Algorithms on Eurocentric Numerical Model Data
[0069] Table 1: Performance comparison of the algorithm with other methods on Eurocentric numerical model data
[0070] method name MAE MPAE Ts 0.1
[0071] .
experiment example 2
[0072] Experimental example 2: Comparison of visualization effects of prediction results
[0073] Figure 4 A comparison of the effects of the generated precipitation forecast maps is shown. Figure 4 The first row is the situation of large-scale precipitation, the second row is the situation of small-scale precipitation; the first column is the forecast map composed of uncorrected numerical model precipitation prediction values, and the second column is the precipitation forecast map of single regression autoencoder , the third column is the result of the precipitation prediction map of the present invention, and the fourth column is the precipitation map composed of the ground observation results. From Figure 4 It can be seen that, no matter in the case of large-scale precipitation or small-scale precipitation, the present invention has more accurate prediction than the uncorrected numerical model, and the effect accuracy is higher than other methods.
[0074] From the a...
PUM
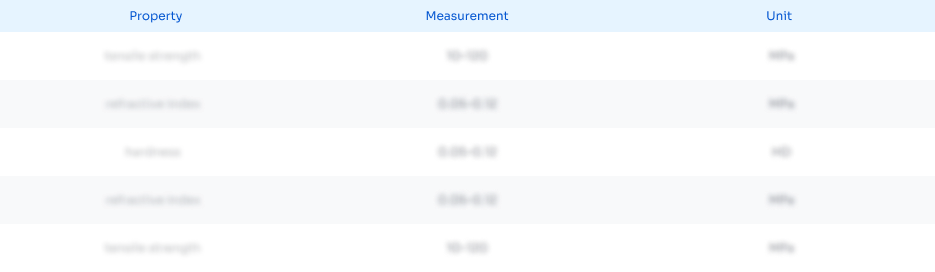
Abstract
Description
Claims
Application Information
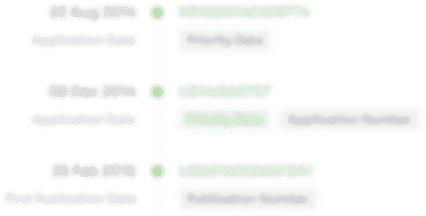
- R&D Engineer
- R&D Manager
- IP Professional
- Industry Leading Data Capabilities
- Powerful AI technology
- Patent DNA Extraction
Browse by: Latest US Patents, China's latest patents, Technical Efficacy Thesaurus, Application Domain, Technology Topic, Popular Technical Reports.
© 2024 PatSnap. All rights reserved.Legal|Privacy policy|Modern Slavery Act Transparency Statement|Sitemap|About US| Contact US: help@patsnap.com