Remote sensing image target detection method based on random access memory
A remote sensing image and target detection technology, applied in image enhancement, image analysis, image data processing, etc., can solve the problems of incomplete fitting of parameter models, difficult to achieve engineering applications, time-consuming and labor-intensive, and achieve good adaptability. and generalization ability, high degree of automation, and the effect of improving efficiency
- Summary
- Abstract
- Description
- Claims
- Application Information
AI Technical Summary
Problems solved by technology
Method used
Image
Examples
Embodiment Construction
[0033] In order to better understand the technical solution of the present invention, the embodiments of the present invention will be further described below in conjunction with the accompanying drawings:
[0034] The present invention is implemented under the framework of Tensorflow, using Python language programming. First complete the network construction and configure relevant parameters; then use the training data to train the network to obtain the tuned network parameters; then build a test model network based on the maximum posterior distribution, use the trained network model and the built The detection network performs object detection on images.
[0035] The SSD network structure prototype based on the present invention is as follows figure 2 As shown, the large cube in front represents the feature extraction layer of VGG16, the convolution layer is a convolution operation on the input data, and the downsampling layer is a maximum pooling operation. In addition, ...
PUM
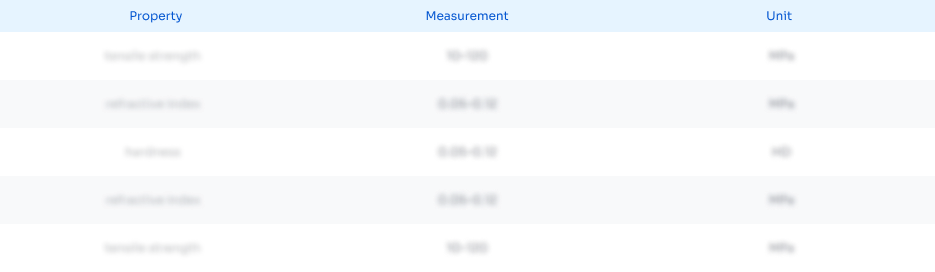
Abstract
Description
Claims
Application Information
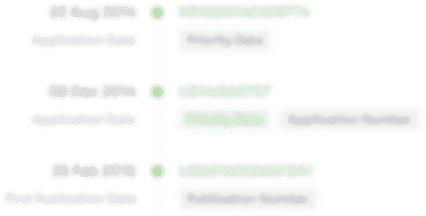
- R&D Engineer
- R&D Manager
- IP Professional
- Industry Leading Data Capabilities
- Powerful AI technology
- Patent DNA Extraction
Browse by: Latest US Patents, China's latest patents, Technical Efficacy Thesaurus, Application Domain, Technology Topic, Popular Technical Reports.
© 2024 PatSnap. All rights reserved.Legal|Privacy policy|Modern Slavery Act Transparency Statement|Sitemap|About US| Contact US: help@patsnap.com