Earthquake motion peak acceleration prediction method based on second-order neuron deep neural network
A deep neural network and peak acceleration technology, applied in neural learning methods, biological neural network models, seismology, etc., can solve problems such as low accuracy of ground motion prediction, and achieve the effects of good applicability, improved accuracy, and high accuracy
- Summary
- Abstract
- Description
- Claims
- Application Information
AI Technical Summary
Problems solved by technology
Method used
Image
Examples
specific Embodiment approach 1
[0038] Specific implementation mode one: the method for predicting the peak acceleration of earthquake motion based on the second-order neuron deep neural network in this implementation mode is implemented according to the following steps:
[0039] Step 1: Collect earthquake records and create a data set:
[0040] Select the magnitude (M), projection distance (R JB ), shear wave velocity (V S30 ), region (Region), cover layer thickness (Z 1 ), fault type (Fault Type) and period (T) are the input parameters, and the corresponding peak acceleration of the ground motion is the target parameter, and the values of the input parameter and the target parameter are between -0.5 and 0.5 through the standardization method, and the ground motion data set;
[0041] Step 2: Build a deep neural network with second-order neurons:
[0042] Establish a deep neural network with three hidden layers. The neurons are all second-order elements. The hyperbolic tangent function (Tanh) is used a...
specific Embodiment approach 2
[0059] Embodiment 2: The difference between this embodiment and Embodiment 1 is that the earthquake records in step 1 are selected from the NGA-West2 database.
specific Embodiment approach 3
[0060] Specific embodiment three: the difference between this embodiment and specific embodiment one or two is that the magnitude (M) in step 1 takes the natural logarithmic value, and the projection distance (R JB ) takes the natural logarithm value.
PUM
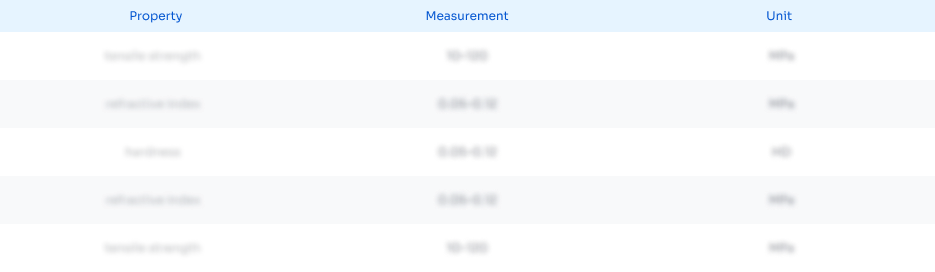
Abstract
Description
Claims
Application Information
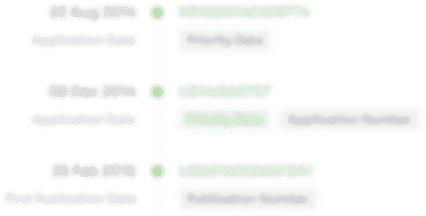
- R&D Engineer
- R&D Manager
- IP Professional
- Industry Leading Data Capabilities
- Powerful AI technology
- Patent DNA Extraction
Browse by: Latest US Patents, China's latest patents, Technical Efficacy Thesaurus, Application Domain, Technology Topic, Popular Technical Reports.
© 2024 PatSnap. All rights reserved.Legal|Privacy policy|Modern Slavery Act Transparency Statement|Sitemap|About US| Contact US: help@patsnap.com