Focus detection model training method based on generative adversarial network
A technology for detecting models and lesions, applied in the computer field, can solve problems such as inability to improve lesion detection performance, unsatisfactory expansion effect, etc.
- Summary
- Abstract
- Description
- Claims
- Application Information
AI Technical Summary
Problems solved by technology
Method used
Image
Examples
Embodiment 1
[0047] The following is an introduction to Embodiment 1 of a training method for a lesion detection model based on a generative adversarial network provided by the present application, see figure 1 , embodiment one includes:
[0048] S101. Obtain an original training sample, where the original training sample includes medical images and labeling information of the target tissue;
[0049] The purpose of this embodiment is to solve the problem of expanding the training samples of the lesion detection model under a small sample size and the unbalanced training samples. This embodiment can be applied to the detection of tumors in various tissues, for example, the detection of tumors in mammography, and the specific detection target can be determined according to the actual application scene. Specifically, a mammography image may be selected as the above medical image, and the detection of the target tissue may be realized based on this.
[0050] Specifically, after obtaining the...
Embodiment 2
[0060] Specifically, Embodiment 2 is mainly used to detect breast masses based on mammography images. see figure 2 , embodiment two specifically includes:
[0061] S201. Obtain an original training sample from a public dataset, where the original training sample includes a mammography target tissue image and labeling information;
[0062] Preferably, S201 includes the following process:
[0063] First, download or cooperate with the hospital to collect a certain amount of desensitized mammography data sets. In the implementation of this embodiment, the INbreast public dataset is used, mainly because this dataset has been extensively researched and used, and it is recognized for its accurate labeling effect, and it is easy to compare the pros and cons of the method with other methods horizontally.
[0064] Then, data cleaning is carried out. Through cleaning, the basic situation of the data set can be understood, and the basic information of the data can be grasped. Prefer...
PUM
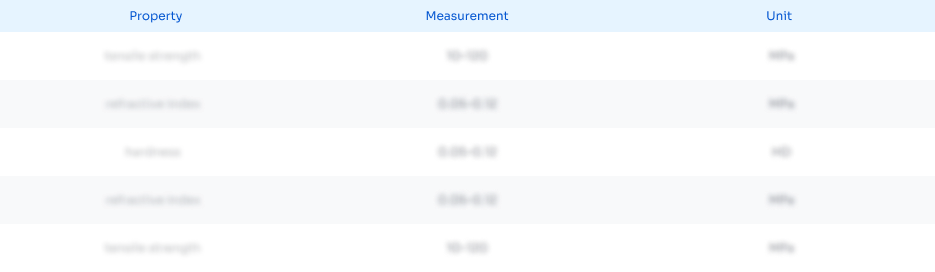
Abstract
Description
Claims
Application Information
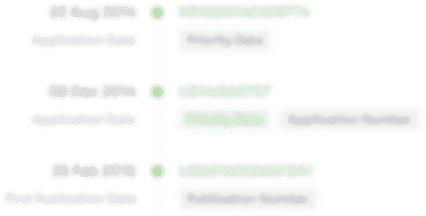
- R&D
- Intellectual Property
- Life Sciences
- Materials
- Tech Scout
- Unparalleled Data Quality
- Higher Quality Content
- 60% Fewer Hallucinations
Browse by: Latest US Patents, China's latest patents, Technical Efficacy Thesaurus, Application Domain, Technology Topic, Popular Technical Reports.
© 2025 PatSnap. All rights reserved.Legal|Privacy policy|Modern Slavery Act Transparency Statement|Sitemap|About US| Contact US: help@patsnap.com