Active power distribution network real-time random optimization scheduling method based on reinforcement learning
An active distribution network and stochastic optimization technology, applied to electrical components, circuit devices, AC network circuits, etc., can solve problems such as slow convergence speed and high initial slope dependence
- Summary
- Abstract
- Description
- Claims
- Application Information
AI Technical Summary
Problems solved by technology
Method used
Image
Examples
Embodiment
[0101] A real-time stochastic optimal scheduling method for active distribution networks based on reinforcement learning, such as figure 1 shown, including the following steps:
[0102] S1. Establish a mathematical model of the active distribution network, and describe the real-time scheduling of the active distribution network as a multi-stage random sequential decision-making problem;
[0103] The mathematical model of the active distribution network includes power flow constraints, energy storage constraints, electric vehicle charging constraints, distributed power supply constraints, and an objective function for real-time scheduling of the active distribution network.
[0104] The power flow constraints are as follows:
[0105]
[0106] In the formula, i and j are node numbers, P ij , Q ij 、r ij and x ij are the active transmission power, reactive transmission power, resistance and reactance between nodes ij, respectively, P i , Q i , V i and δ i are the activ...
PUM
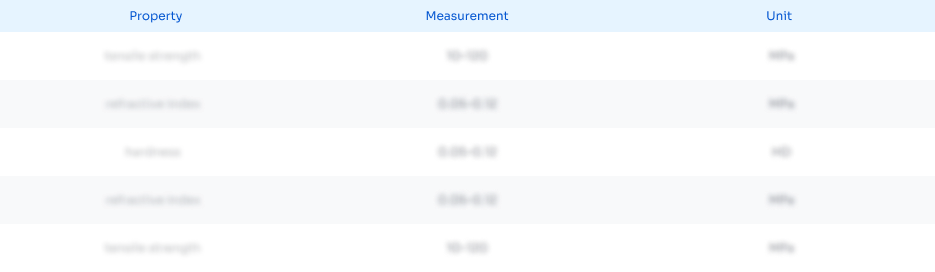
Abstract
Description
Claims
Application Information
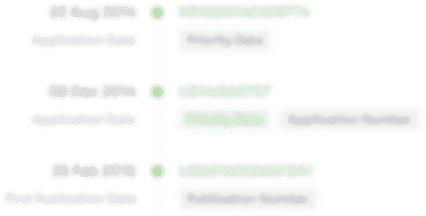
- R&D Engineer
- R&D Manager
- IP Professional
- Industry Leading Data Capabilities
- Powerful AI technology
- Patent DNA Extraction
Browse by: Latest US Patents, China's latest patents, Technical Efficacy Thesaurus, Application Domain, Technology Topic.
© 2024 PatSnap. All rights reserved.Legal|Privacy policy|Modern Slavery Act Transparency Statement|Sitemap