Random asymptotic photon mapping image denoising method and system based on neural network
A neural network and photon mapping technology, used in image enhancement, image analysis, image data processing, etc., can solve the problem of increasing the difficulty of noise reduction, and achieve the effect of increasing stability, maintaining lighting details, and ensuring quality.
- Summary
- Abstract
- Description
- Claims
- Application Information
AI Technical Summary
Problems solved by technology
Method used
Image
Examples
Embodiment 1
[0039] Embodiment 1, this embodiment provides a neural network-based random asymptotic photon mapping image noise reduction method;
[0040] A random asymptotic photon mapping image noise reduction method based on neural network, including:
[0041] S100: Acquire a three-dimensional scene, and generate an image to be denoised based on random progressive photon mapping, where the image to be denoised includes a rendered image based on global photons and a rendered image based on caustic photons;
[0042] S200: Input the rendered image based on global photons into the pre-trained first multiple residual neural network, and output the global photon noise reduction image; input the rendered image based on caustic photons into the pre-trained second multiple In the residual neural network, the caustic photon noise reduction image is output;
[0043] S300: Combining the global photon noise reduction image and the caustic photon noise reduction image to obtain a final rendered image...
Embodiment 2
[0116] Embodiment 2, this embodiment provides a neural network-based random asymptotic photon mapping image noise reduction system;
[0117] A neural network based stochastic asymptotic photon mapping image denoising system, including:
[0118] A data generation module configured to: acquire a three-dimensional scene, generate an image to be denoised based on random progressive photon mapping, the image to be denoised includes a rendered image based on global photons and a rendered image based on caustic photons;
[0119] The noise reduction module is configured to: input the rendered image based on the global photon into the pre-trained first multiple residual neural network, and output the global photon noise reduction image; input the rendered image based on the caustic photon into In the pre-trained second multiple residual neural network, the caustic photon noise reduction image is output;
[0120] The synthesis module is configured to: synthesize the global photon noise...
Embodiment 3
[0122] Embodiment 3. This embodiment also provides an electronic device, including a memory, a processor, and computer instructions stored in the memory and run on the processor. When the computer instructions are executed by the processor, the computer instructions in Embodiment 1 are completed. described method.
[0123] It should be understood that in this embodiment, the processor can be a central processing unit CPU, and the processor can also be other general-purpose processors, digital signal processors DSP, application specific integrated circuits ASIC, off-the-shelf programmable gate array FPGA or other programmable logic devices , discrete gate or transistor logic devices, discrete hardware components, etc. A general-purpose processor may be a microprocessor, or the processor may be any conventional processor, or the like.
[0124] The memory may include read-only memory and random access memory, and provides instructions and data to the processor, and a part of the...
PUM
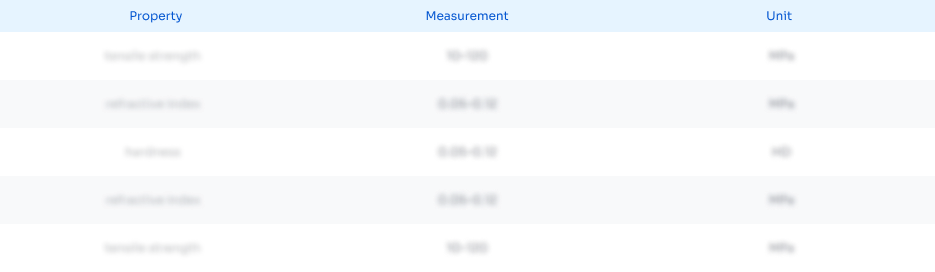
Abstract
Description
Claims
Application Information
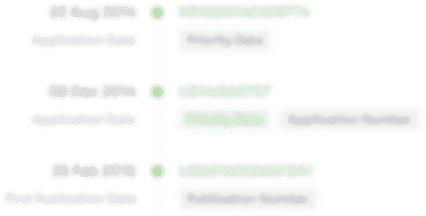
- R&D Engineer
- R&D Manager
- IP Professional
- Industry Leading Data Capabilities
- Powerful AI technology
- Patent DNA Extraction
Browse by: Latest US Patents, China's latest patents, Technical Efficacy Thesaurus, Application Domain, Technology Topic, Popular Technical Reports.
© 2024 PatSnap. All rights reserved.Legal|Privacy policy|Modern Slavery Act Transparency Statement|Sitemap|About US| Contact US: help@patsnap.com