Novel One-Shot learning method based on SimpleShot
A new method and base class technology, applied in the field of target recognition technology for small-sample learning, can solve problems such as over-fitting, achieve the effects of improving accuracy, alleviating over-fitting problems, and improving classification accuracy
- Summary
- Abstract
- Description
- Claims
- Application Information
AI Technical Summary
Problems solved by technology
Method used
Image
Examples
Embodiment Construction
[0029] The technical scheme of the present invention will be further described below in conjunction with the accompanying drawings.
[0030] Such as figure 1 As shown, the present invention provides a small sample learning method based on SimpleShot, mainly for one-shot learning, including the following steps:
[0031] S100: Suppose we get a data set D formed by a base class base ={(I 1 ,y 1 ),..., (I N ,y N ))}, which contains N labeled images from the base class A, namely y n ∈{1,...,A},I N Indicates the Nth image, y N For the label of the Nth image, use EfficientNets as the feature extraction network f θ After training on this base class, this trained model is called feature space;
[0032] The implementation of further step S100 is as follows:
[0033] Using the base class to train the feature network is carried out in the normal steps of the usual image classification, as follows:
[0034] Suppose we get a data set D base ={(I 1 ,y 1 ),..., (I N ,y N ))}, ...
PUM
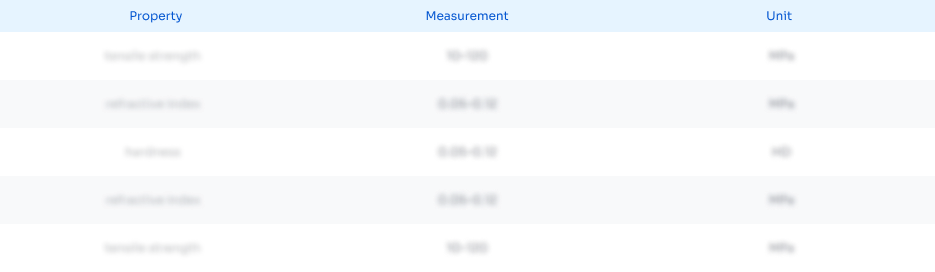
Abstract
Description
Claims
Application Information
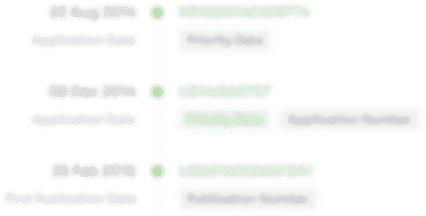
- R&D Engineer
- R&D Manager
- IP Professional
- Industry Leading Data Capabilities
- Powerful AI technology
- Patent DNA Extraction
Browse by: Latest US Patents, China's latest patents, Technical Efficacy Thesaurus, Application Domain, Technology Topic.
© 2024 PatSnap. All rights reserved.Legal|Privacy policy|Modern Slavery Act Transparency Statement|Sitemap