Soybean and corn planting area identification method in Jianghuai region based on Sentinel-2 image
A recognition method, soybean technology, applied in the field of crop image recognition, can solve problems such as complex planting structure, high proportion of satellite image cloud pollution, and increased difficulty in mapping
- Summary
- Abstract
- Description
- Claims
- Application Information
AI Technical Summary
Problems solved by technology
Method used
Image
Examples
Embodiment 2
[0035] Embodiment 2, in the described step C, include the following steps: C11, extract a plurality of quadrat images with a set size from random positions in the satellite image, including soybeans, corn, sorghum, buildings, forests, roads in the quadrat area , water body, bare land, and other vegetation, a total of nine land cover types; C12, obtain the high-definition image of the corresponding position of the sample plot from the high-definition image taken by the drone equipped with a high-definition camera and Google Earth; C13, artificially mark the high-definition image of the corresponding position of the sample plot The land cover type in the image; C14. Correspond the marked land cover type to the satellite image of the original quadrat according to the coordinate position to form a training set; C15. Combine the training set data, 20 features, and the set decision The number of tree numbers and the number of feature variables used in each split node are substituted ...
Embodiment 3
[0037] Embodiment three, in the described step C, include the following steps: C21, execute the steps C11-C14 in claim 6 to obtain the training set; C22, calculate the importance score of twenty features in the random forest algorithm; C23, According to the scores of the features from high to low, add twenty features to the random forest classifier in turn, build a classification model by sequential forward selection, and then verify the classification accuracy of different models to determine the number of features in the best feature set; C24, Substituting the training set data, the features in the best feature set, the set number of decision trees and the number of feature variables used in each split node into the random forest algorithm to train the second random forest algorithm model; C25. The image in step B is substituted into the second random forest algorithm model to realize the recognition of each pixel in the image. The more features, the longer the training time...
PUM
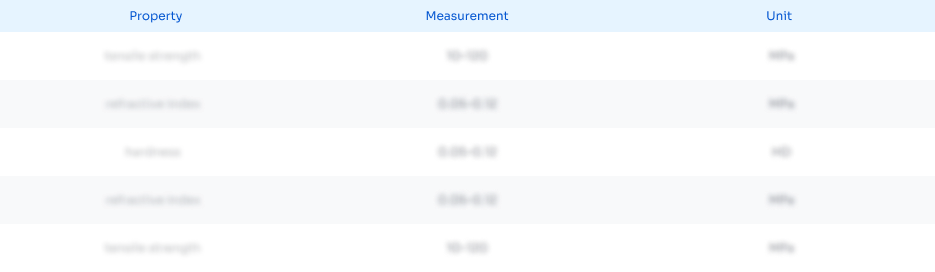
Abstract
Description
Claims
Application Information
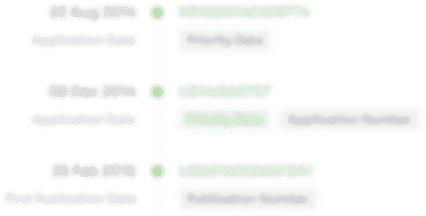
- R&D Engineer
- R&D Manager
- IP Professional
- Industry Leading Data Capabilities
- Powerful AI technology
- Patent DNA Extraction
Browse by: Latest US Patents, China's latest patents, Technical Efficacy Thesaurus, Application Domain, Technology Topic, Popular Technical Reports.
© 2024 PatSnap. All rights reserved.Legal|Privacy policy|Modern Slavery Act Transparency Statement|Sitemap|About US| Contact US: help@patsnap.com