Remote sensing image semantic segmentation method combining deep learning and random forest
A semantic segmentation and random forest technology, applied in neural learning methods, computer parts, character and pattern recognition, etc., can solve the problems of low segmentation accuracy and limited versatility of methods, so as to improve classification accuracy, improve efficiency and universality. Sex, the effect of less bands
- Summary
- Abstract
- Description
- Claims
- Application Information
AI Technical Summary
Problems solved by technology
Method used
Image
Examples
Embodiment 1
[0065] as attached figure 1 As shown, a remote sensing image semantic segmentation method combining deep learning and random forest includes the following steps:
[0066] 101. Create a training data set for the research area, using samples and sample labels as the training data set.
[0067] Acquisition of samples and sample labels: First obtain the GF-2 image, synthesize the red, green and blue bands of the image, and crop the composite image into a sample. The cropping specification is 512*512, as attached figure 2 As shown, mark on the labelme software, as attached image 3 As shown, the saved data is a json file, and the json file is converted into a dataset file. At this time, the label data is 8-bit depth label data, and finally it is given "true color" and converted into a 24-bit depth sample label file. as attached Figure 4 shown.
[0068] The training data set in the research area uses 512*512*24 bit depth samples and sample labels as the specifications of the t...
Embodiment 2
[0109] Based on the above-mentioned embodiment 1, as attached Figure 5 As shown, a GF-2 optical image data is used as the data source for the data of the study area. The samples and sample labels in the above-mentioned embodiment 1 are used as the training data set.
[0110] Use the Python language to write a fully convolutional neural network model based on the TensorFlow framework, use the above samples and sample labels to train the model, use the trained model to test the data in the research area, extract its deep features, and visualize it.
[0111] Use the Band Math function of ENVI5.3 software to extract the normalized vegetation index and normalized water index of GF-2 image data according to the formula (3) and formula (4); the Band Math function is the pixel value of each pixel Perform numerical operations.
[0112] Use the Co-occurrence Measures function of ENVI5.3 software to extract the variance, entropy, dissimilarity and angular second-order moment of four t...
PUM
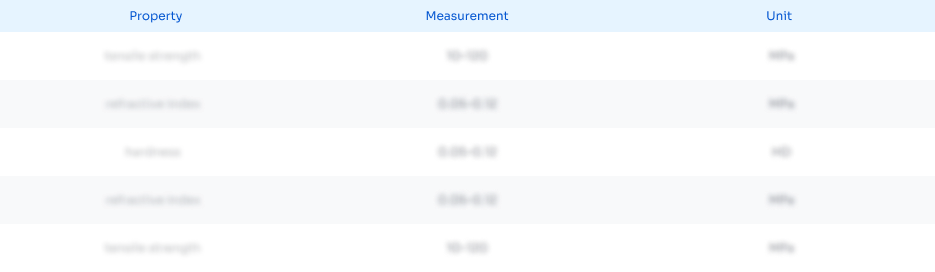
Abstract
Description
Claims
Application Information
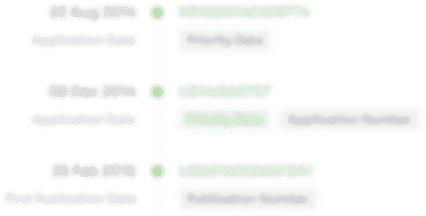
- R&D Engineer
- R&D Manager
- IP Professional
- Industry Leading Data Capabilities
- Powerful AI technology
- Patent DNA Extraction
Browse by: Latest US Patents, China's latest patents, Technical Efficacy Thesaurus, Application Domain, Technology Topic.
© 2024 PatSnap. All rights reserved.Legal|Privacy policy|Modern Slavery Act Transparency Statement|Sitemap