GAN-based speech confrontation sample generation method
A technology against samples and samples, applied in speech analysis, neural learning methods, biological neural network models, etc., can solve the problems of target speech recognition network recognition errors, no guarantee of generated speech quality, and inability to guarantee the speech quality of confrontation samples, etc., to achieve Improve efficiency and ensure voice quality
- Summary
- Abstract
- Description
- Claims
- Application Information
AI Technical Summary
Problems solved by technology
Method used
Image
Examples
Embodiment Construction
[0034] Embodiments of the present invention are described in detail below, examples of which are shown in the drawings, wherein the same or similar reference numerals designate the same or similar elements or elements having the same or similar functions throughout. The embodiments described below by referring to the figures are exemplary only for explaining the present invention and should not be construed as limiting the present invention.
[0035] Such as Figures 1 to 3 Shown is a schematic structural diagram of a preferred embodiment of the present invention. The present invention adopts a GAN-based voice confrontation sample generation method, utilizes the game idea of generative confrontation network, and designs a reasonable loss function to train a disturbance generator, through which the disturbance generator can quickly construct a voice with better quality and a successful attack Speech adversarial samples with high rate. Such as figure 1 Shown is a diagram of...
PUM
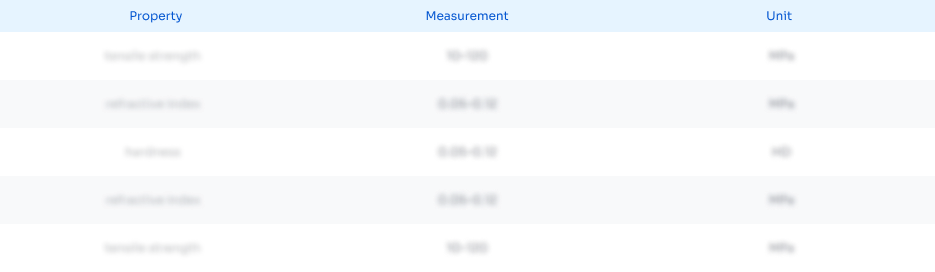
Abstract
Description
Claims
Application Information
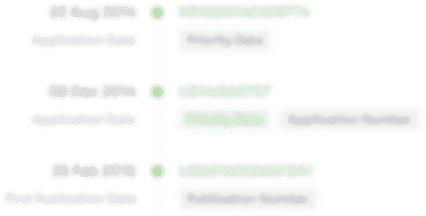
- R&D Engineer
- R&D Manager
- IP Professional
- Industry Leading Data Capabilities
- Powerful AI technology
- Patent DNA Extraction
Browse by: Latest US Patents, China's latest patents, Technical Efficacy Thesaurus, Application Domain, Technology Topic, Popular Technical Reports.
© 2024 PatSnap. All rights reserved.Legal|Privacy policy|Modern Slavery Act Transparency Statement|Sitemap|About US| Contact US: help@patsnap.com