Large-scale experimental device power device fault diagnosis method based on deep convolutional neural network
A neural network model, deep convolution technology, applied in biological neural network models, neural learning methods, measurement devices, etc., to achieve the effect of improving accuracy, simple data collection methods, and accurate conclusions
- Summary
- Abstract
- Description
- Claims
- Application Information
AI Technical Summary
Problems solved by technology
Method used
Image
Examples
Embodiment
[0061] In this embodiment, 80% of the normalized sample set is used as a training set, and 20% is used as a test set. The method proposed by the invention and the designed artificial intelligence algorithm model are used to accurately diagnose the fault type of the electric equipment; the correct rate of fault diagnosis of the electric equipment can reach more than 90%.
[0062] By adopting the above-mentioned technical scheme disclosed by the present invention, the following beneficial effects are obtained:
[0063] The invention discloses a large-scale experimental device fault diagnosis method for electric equipment based on a deep convolutional neural network. A fault diagnosis model is established according to the working data of the working state of the equipment, and the hidden information of the working data is deeply excavated through the fault diagnosis model, and The hyperparameters of the fault diagnosis model are adjusted according to the feedback test results, th...
PUM
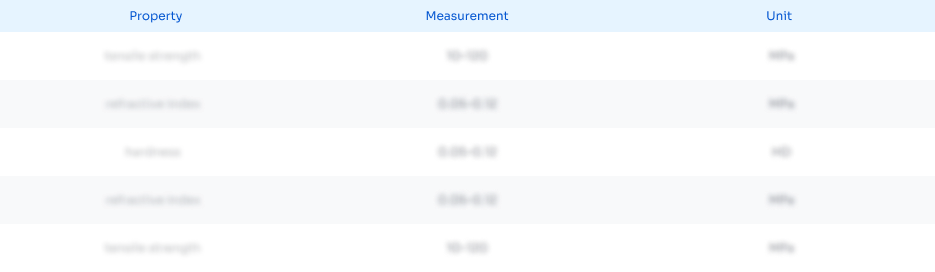
Abstract
Description
Claims
Application Information
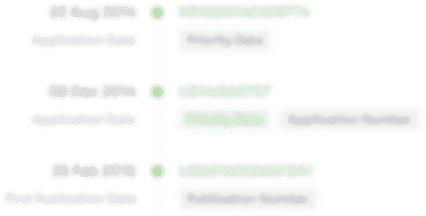
- R&D
- Intellectual Property
- Life Sciences
- Materials
- Tech Scout
- Unparalleled Data Quality
- Higher Quality Content
- 60% Fewer Hallucinations
Browse by: Latest US Patents, China's latest patents, Technical Efficacy Thesaurus, Application Domain, Technology Topic, Popular Technical Reports.
© 2025 PatSnap. All rights reserved.Legal|Privacy policy|Modern Slavery Act Transparency Statement|Sitemap|About US| Contact US: help@patsnap.com