Multi-source atmospheric data clustering method based on distribution density
A technology of distribution density and atmospheric data, applied in other database clustering/classification, other database indexing, other database retrieval, etc., can solve the problem of inability to distinguish noise points, unallocated points allocation accuracy is not high, and cluster centers are difficult to accurately identify, etc. question
- Summary
- Abstract
- Description
- Claims
- Application Information
AI Technical Summary
Problems solved by technology
Method used
Image
Examples
Embodiment Construction
[0063] The present invention will be described in further detail below in conjunction with the accompanying drawings.
[0064] The distribution density is in a data set DS composed of M-dimensional data with a data volume of N, the distribution density dd(i,j) of any item of data (vertex) i to another item of data j is:
[0065]
[0066] Where V(i,k) represents the hypersphere volume with the vertex i as the center and the distance from i to k as the radius, DS(i,j) represents the vertices within the range of V(i,j), PN(i,j ) represents the number of vertices within the range of V(i,j), and the hypersphere formula is as follows:
[0067]
[0068] where r is the radius, M is the data dimension, and Γ is the gamma function.
[0069] The above formula (1) for defining the distribution density expresses the ratio of the number of vertices in the hypersphere formed by the distance between any two vertices in the data set as the radius to the sum of the volume of the hypersph...
PUM
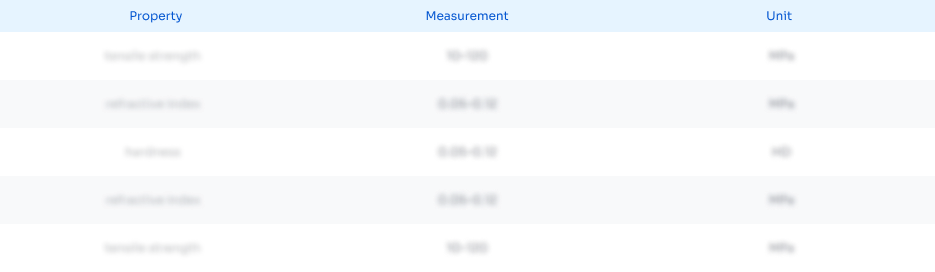
Abstract
Description
Claims
Application Information
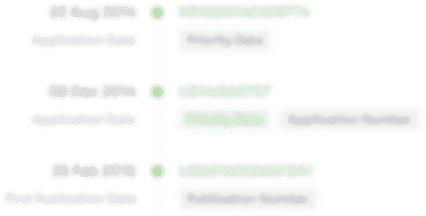
- R&D Engineer
- R&D Manager
- IP Professional
- Industry Leading Data Capabilities
- Powerful AI technology
- Patent DNA Extraction
Browse by: Latest US Patents, China's latest patents, Technical Efficacy Thesaurus, Application Domain, Technology Topic, Popular Technical Reports.
© 2024 PatSnap. All rights reserved.Legal|Privacy policy|Modern Slavery Act Transparency Statement|Sitemap|About US| Contact US: help@patsnap.com