Target tracking method and training method based on matching-regression network
A matching network and target tracking technology, applied in the field of target tracking and training based on matching-regression network, can solve the problems of less prior knowledge of tracking target, poor ability to adapt to target deformation, and no use of video sequence timing information, etc. Effects of Accuracy and Robustness
- Summary
- Abstract
- Description
- Claims
- Application Information
AI Technical Summary
Problems solved by technology
Method used
Image
Examples
Embodiment
[0069] In this embodiment, the PyTorch deep learning framework is used to realize the network in this method, and the machines used for training and testing are equipped with Intel i7-7800X CPU, 32G memory and two NVIDIA GTX1080 Ti GPUs. The data sets used for the evaluation are VOT2016 and VOT2018, and the evaluation tool is VOT toolkit. The method flow is attached image 3 And attached Figure 4 shown.
[0070] Both the VOT2016 and VOT2018 datasets contain 60 video sequences, and each frame in the sequence is labeled with attributes, which can determine whether the image illumination has changed, whether the camera has moved, whether the target shape, size, and moving direction have changed, and whether the target has been changed. Obstruction by other objects, or other situations. This embodiment mainly uses the accuracy, robustness and EAO value in the VOT competition to evaluate the tracking algorithm. Accuracy represents the average overlapping area ratio between the...
PUM
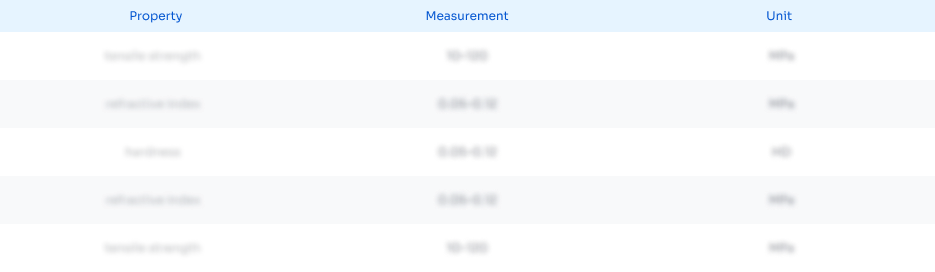
Abstract
Description
Claims
Application Information
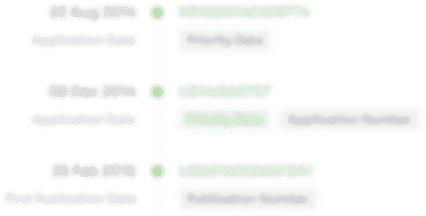
- R&D Engineer
- R&D Manager
- IP Professional
- Industry Leading Data Capabilities
- Powerful AI technology
- Patent DNA Extraction
Browse by: Latest US Patents, China's latest patents, Technical Efficacy Thesaurus, Application Domain, Technology Topic, Popular Technical Reports.
© 2024 PatSnap. All rights reserved.Legal|Privacy policy|Modern Slavery Act Transparency Statement|Sitemap|About US| Contact US: help@patsnap.com