Fatigue detection method and device based on GPDC graph convolutional neural network and storage medium
A convolutional neural network and fatigue detection technology, applied in neural learning methods, biological neural network models, neural architectures, etc., can solve problems such as manual extraction, complex and cumbersome operations, irregularities, etc., and achieve the effect of improving detection performance
- Summary
- Abstract
- Description
- Claims
- Application Information
AI Technical Summary
Problems solved by technology
Method used
Image
Examples
Embodiment 1
[0053] Reference figure 1 , Embodiment 1 of the present invention provides a fatigue detection method based on GPDC graph convolutional neural network, and one of the embodiments includes but is not limited to the following steps:
[0054] Step S100, collecting initial brain electricity data.
[0055] In this embodiment, the initial EEG data is collected in this step to prepare for the subsequent driving fatigue detection and provide a data basis.
[0056] In step S200, the initial EEG data is processed to obtain a first EEG signal.
[0057] In this embodiment, this step organizes and processes the collected initial EEG data to further prepare for driving fatigue detection.
[0058] In step S300, the first EEG signal is segmented and a directed static network is calculated through generalized biased coherence.
[0059] In this embodiment, the generalized biased coherence (GPDC) in this step is a frequency-domain causal analysis method of multi-channel EEG signals. The causal relationshi...
Embodiment 2
[0124] Reference image 3 The second embodiment of the present invention provides a fatigue detection device 1000 based on a GPDC graph convolutional neural network, including:
[0125] The collecting unit 1100 is used to collect initial brain electricity data;
[0126] The processing unit 1200 is configured to process the initial EEG data to obtain a first EEG signal;
[0127] The operating unit 1300 is configured to segment the first EEG signal and calculate a directed static network through generalized bias coherent calculation;
[0128] The screening processing unit 1400 is configured to screen out important connection information from the directed static network, and obtain a brain function matrix according to the important connection information;
[0129] The establishment unit 1500 is configured to establish a directed brain function network through the brain function matrix;
[0130] A modeling unit 1600, configured to model the extracted EEG features into a graph signal through ...
Embodiment 3
[0141] The third embodiment of the present invention also provides a computer-readable storage medium that stores executable instructions of a fatigue detection device based on a GPDC graph convolutional neural network, and fatigue detection based on a GPDC graph convolutional neural network The device executable instructions are used to make the fatigue detection device based on the GPDC graph convolutional neural network execute the above-mentioned fatigue detection method based on the GPDC graph convolutional neural network, for example, execute the above-described fatigue detection method figure 1 Steps S100 to S800 in the method to achieve image 3 The functions of the units 1000-1800 in.
PUM
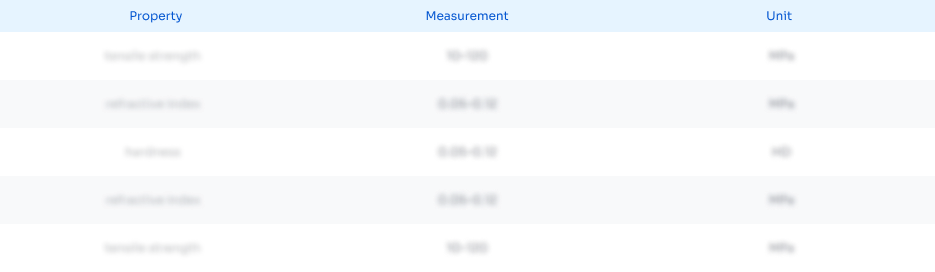
Abstract
Description
Claims
Application Information
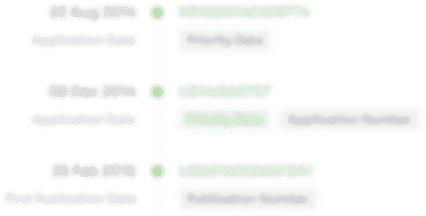
- R&D
- Intellectual Property
- Life Sciences
- Materials
- Tech Scout
- Unparalleled Data Quality
- Higher Quality Content
- 60% Fewer Hallucinations
Browse by: Latest US Patents, China's latest patents, Technical Efficacy Thesaurus, Application Domain, Technology Topic, Popular Technical Reports.
© 2025 PatSnap. All rights reserved.Legal|Privacy policy|Modern Slavery Act Transparency Statement|Sitemap|About US| Contact US: help@patsnap.com