Wind turbine generator gearbox fault diagnosis method based on wavelet packet decomposition and convolutional neural network
A convolutional neural network and wavelet packet decomposition technology, applied in neural learning methods, biological neural network models, neural architectures, etc., can solve problems such as limited recognition effect, not end-to-end, and lack of multi-scale features of learning signals, and achieve Avoid the loss of useful information and have good versatility
- Summary
- Abstract
- Description
- Claims
- Application Information
AI Technical Summary
Problems solved by technology
Method used
Image
Examples
Embodiment Construction
[0050] In order to make the technical scheme and design idea of the present invention clearer, we describe it in detail in conjunction with the accompanying drawings.
[0051] figure 1 It is a framework flowchart of the method of the present invention, and the implementation process is divided into a training process and a real-time fault diagnosis process. Among them, WPD layer is the wavelet packet decomposition layer, which performs wavelet packet decomposition on the data, corresponding to step 2; C1 layer is the first convolutional layer, P1 layer is the first pooling layer, and C2 layer is the second Layer convolution layer, P2 layer is the second pooling layer, these layers are responsible for the convolution pooling step, corresponding to step 3; FC layer is a fully connected layer, S layer is a Softmax layer, responsible for classification results, corresponding to step 4.
[0052] Training requires labeling the data and certain deep learning parameter tuning skill...
PUM
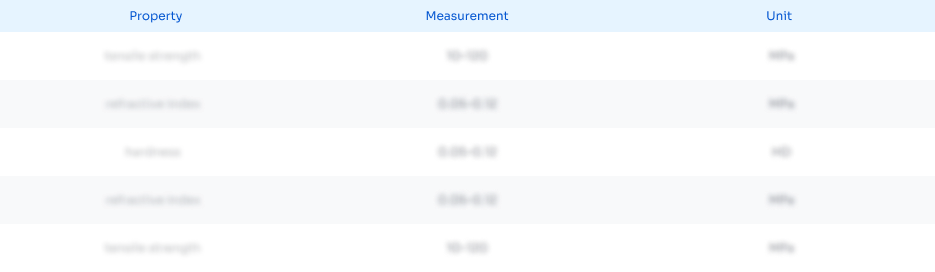
Abstract
Description
Claims
Application Information
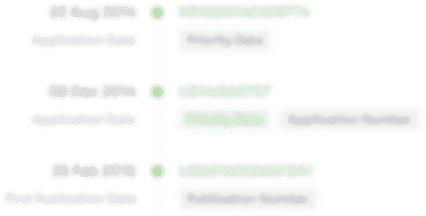
- R&D Engineer
- R&D Manager
- IP Professional
- Industry Leading Data Capabilities
- Powerful AI technology
- Patent DNA Extraction
Browse by: Latest US Patents, China's latest patents, Technical Efficacy Thesaurus, Application Domain, Technology Topic.
© 2024 PatSnap. All rights reserved.Legal|Privacy policy|Modern Slavery Act Transparency Statement|Sitemap