Method for classifying electrocardiosignals by using optimized AdaBoost weighting mode and weak classifier
A weak classifier, electrocardiographic signal technology, applied in the fields of instruments, character and pattern recognition, computer parts, etc., can solve the problems of weak classifiers without considering the wrong sample misclassification rate, low accuracy, etc., to improve the screening method, Good classification, obvious effect of classification
- Summary
- Abstract
- Description
- Claims
- Application Information
AI Technical Summary
Problems solved by technology
Method used
Image
Examples
Embodiment 1
[0039] Such as figure 1 , figure 2 Shown, a kind of method utilizing optimized AdaBoost weighting mode and weak classifier to ECG signal classification, described method comprises:
[0040] S1: Obtain ECG data, the data set comes from the arrhythmia database in the MIT-BIH database (the database for researching arrhythmia provided by the Massachusetts Institute of Technology);
[0041] S2: Carry out ECG data feature extraction, described feature extraction algorithm is discrete wavelet transform (DWT), is specifically binary wavelet transform, concrete steps are as follows:
[0042] S201: Take the first 500 sampling points to ensure the data volume of the preprocessed ECG signal;
[0043] S202: Set the positive threshold to be 1 / 4 of the average value of the maximum value point amplitude, and the negative threshold value to be 1 / 4 of the average value of the minimum value point amplitude value;
[0044] S203: The function selects a quadratic B-spline wavelet with a scale o...
Embodiment 2
[0063] This embodiment verifies the improved algorithm.
[0064] Binary classification algorithms often use the ROC (Receiver Operating Characteristic) curve and AUC (Area Under the Curve) value to judge the performance of the classifier; the specific process is: divide the two types of samples into positive and negative, and use the model The correctly classified samples are denoted by TP, the positive class misclassified samples are denoted by FP; the negative class misclassified samples are denoted by TN, and the negative class misclassified samples are denoted by FN. Such as Figure 4 As shown, the abscissa of the curve is False positive rate (FPR), and the ordinate is True positive rate (TPR). The AUC value refers to the area enclosed by the ROC curve and the false positive rate (FPR) on the abscissa.
[0065]
[0066]
PUM
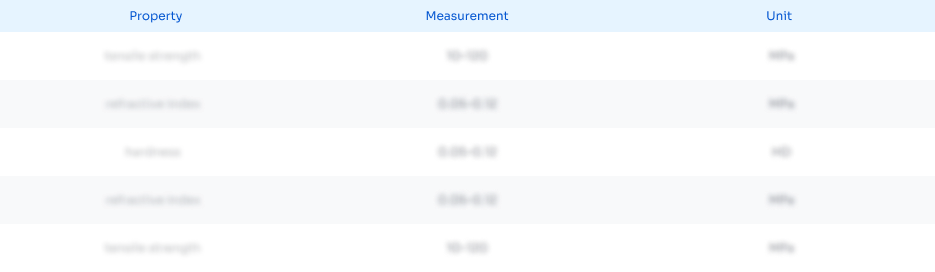
Abstract
Description
Claims
Application Information
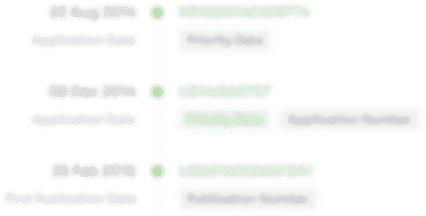
- R&D
- Intellectual Property
- Life Sciences
- Materials
- Tech Scout
- Unparalleled Data Quality
- Higher Quality Content
- 60% Fewer Hallucinations
Browse by: Latest US Patents, China's latest patents, Technical Efficacy Thesaurus, Application Domain, Technology Topic, Popular Technical Reports.
© 2025 PatSnap. All rights reserved.Legal|Privacy policy|Modern Slavery Act Transparency Statement|Sitemap|About US| Contact US: help@patsnap.com