Lung lobe segmentation method and system based on three-dimensional convolutional neural network
A neural network and three-dimensional convolution technology, applied in the field of image processing, can solve problems such as unclear boundaries of watershed, large amount of calculation, and unrealized workflow, so as to achieve accurate positioning of lung lobe boundaries, improve model robustness, easy implementation and The effect of deployment
- Summary
- Abstract
- Description
- Claims
- Application Information
AI Technical Summary
Problems solved by technology
Method used
Image
Examples
Embodiment
[0068] Such as figure 1 As shown, this embodiment provides a lung lobe segmentation method based on a three-dimensional convolutional neural network, which realizes end-to-end lung lobe segmentation and improves the efficiency and accuracy of lung lobe segmentation. The specific steps include:
[0069] (1) In the data set construction stage, construct a lung lobe segmentation data set for neural network training;
[0070] Specific steps include:
[0071] (1-1) Use Materialize Mimics 22.0 software to label the lung lobe images. The lung lobe segmentation data set in this embodiment is pixel-level labeling. The labeling content includes: right lung, left lung, right upper lobe, right middle lobe, right lower lobe, Left upper lobe and left lower lobe. In this example, a total of 100 cases of data are annotated. The data source is taken from the LUNA16 data set. The LUNA16 data set includes 888 low-dose lung CT image data, and each image contains a series of multiple axial slices...
PUM
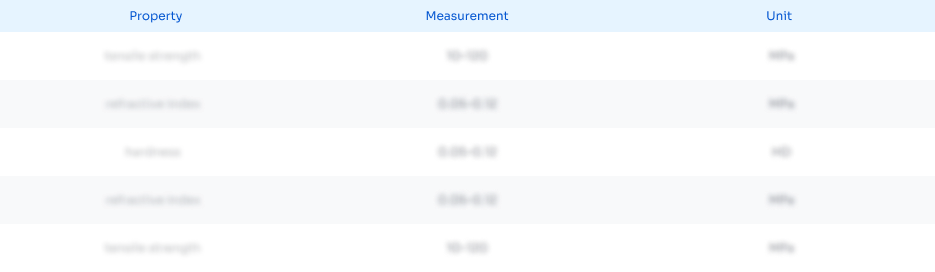
Abstract
Description
Claims
Application Information
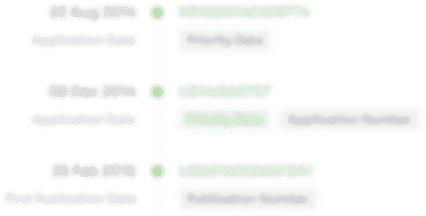
- R&D Engineer
- R&D Manager
- IP Professional
- Industry Leading Data Capabilities
- Powerful AI technology
- Patent DNA Extraction
Browse by: Latest US Patents, China's latest patents, Technical Efficacy Thesaurus, Application Domain, Technology Topic, Popular Technical Reports.
© 2024 PatSnap. All rights reserved.Legal|Privacy policy|Modern Slavery Act Transparency Statement|Sitemap|About US| Contact US: help@patsnap.com