Low-rank sparse decomposition hyperspectral anomaly detection method based on local features
A local feature, sparse decomposition technology, applied in measurement devices, analysis materials, image analysis and other directions, can solve problems such as affecting the detection accuracy of abnormal targets, insufficient low-rank sparse matrix decomposition accuracy, etc., to improve interpretability, easy to implement, The effect of fewer parameters
- Summary
- Abstract
- Description
- Claims
- Application Information
AI Technical Summary
Problems solved by technology
Method used
Image
Examples
Embodiment Construction
[0047] The key invention of the present invention is a low-rank sparse matrix decomposition hyperspectral abnormal target detection method based on local features, which solves the problem of lack of image-based local feature information in traditional methods. Combine below figure 1 , the specific embodiment of the present invention will be described in further detail.
[0048] Based on the image matrix X, abnormal target detection is performed on hyperspectral remote sensing images. The specific implementation process is as follows:
[0049] Read the original 3D hyperspectral image into a matrix X={x 1 ,...,x t ,...,x i}, i is the number of image bands, and j is the number of image pixels. Each column of vector x in matrix X j =(x1j ,...,x tj ,...,x ij ) T is the spectral radiance value of the pixel. Perform the following operations on the hyperspectral remote sensing image matrix X:
[0050] (1) Construct a hyperspectral image description model based on local feat...
PUM
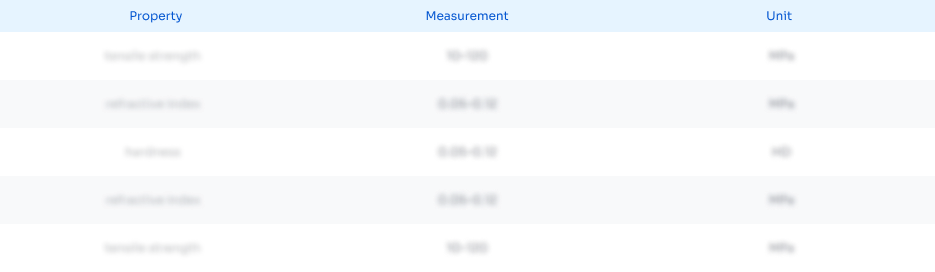
Abstract
Description
Claims
Application Information
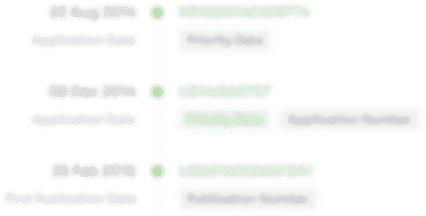
- R&D
- Intellectual Property
- Life Sciences
- Materials
- Tech Scout
- Unparalleled Data Quality
- Higher Quality Content
- 60% Fewer Hallucinations
Browse by: Latest US Patents, China's latest patents, Technical Efficacy Thesaurus, Application Domain, Technology Topic, Popular Technical Reports.
© 2025 PatSnap. All rights reserved.Legal|Privacy policy|Modern Slavery Act Transparency Statement|Sitemap|About US| Contact US: help@patsnap.com