Virtual optical network mapping method based on multi-agent deep reinforcement learning
A virtual optical network and reinforcement learning technology, applied in neural learning methods, data exchange networks, biological neural network models, etc., can solve the problems of high blocking rate and low network utilization.
- Summary
- Abstract
- Description
- Claims
- Application Information
AI Technical Summary
Problems solved by technology
Method used
Image
Examples
Embodiment Construction
[0065] The following will clearly and completely describe the technical solutions in the embodiments of the present invention with reference to the accompanying drawings in the embodiments of the present invention. Obviously, the described embodiments are only some, not all, embodiments of the present invention. Based on the embodiments of the present invention, all other embodiments obtained by persons of ordinary skill in the art without making creative efforts belong to the protection scope of the present invention.
[0066] Such as figure 1 As shown, a virtual optical network mapping method based on multi-agent deep reinforcement learning, the steps are:
[0067] Step 1: Execute the underlying physical network resource scheduling update algorithm, and request the arrival time T of service R in the virtual network a , to judge whether there are other virtual network services leaving the underlying physical network in the environment module at this moment. If so, the enviro...
PUM
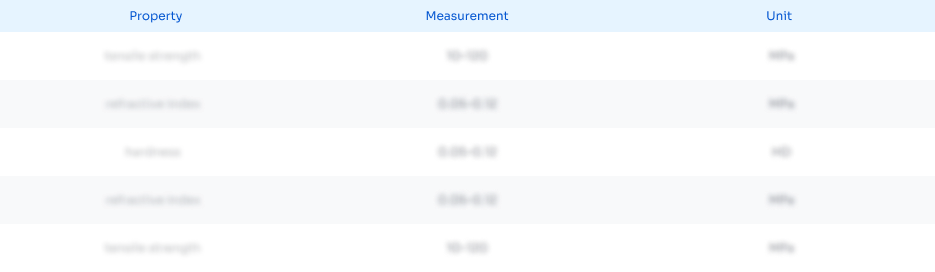
Abstract
Description
Claims
Application Information
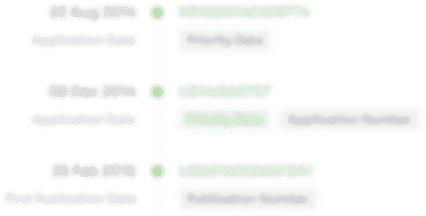
- Generate Ideas
- Intellectual Property
- Life Sciences
- Materials
- Tech Scout
- Unparalleled Data Quality
- Higher Quality Content
- 60% Fewer Hallucinations
Browse by: Latest US Patents, China's latest patents, Technical Efficacy Thesaurus, Application Domain, Technology Topic, Popular Technical Reports.
© 2025 PatSnap. All rights reserved.Legal|Privacy policy|Modern Slavery Act Transparency Statement|Sitemap|About US| Contact US: help@patsnap.com