Compression method and compression device of neural network model, storage medium and equipment
A compression method and convolutional neural network technology, applied in biological neural network models, neural learning methods, neural architectures, etc., can solve problems such as incomplete network models, excessive compression of convolutional layers, and low model accuracy, and improve Operation speed, high accuracy, and the effect of ensuring integrity
- Summary
- Abstract
- Description
- Claims
- Application Information
AI Technical Summary
Problems solved by technology
Method used
Image
Examples
Embodiment Construction
[0067] In order to make the objectives, technical solutions and advantages of the present invention clearer, the following further describes the present invention in detail with reference to the accompanying drawings and embodiments. It should be understood that the specific embodiments described herein are only used to explain the present invention, but not to limit the present invention.
[0068] Before describing the technical solution of the present invention in detail, first briefly describe the inventive concept of the present application: the compression method of the present application combines migration learning and compression algorithms, that is, compressing while migrating, achieving complementary advantages, while ensuring a higher accuracy rate. , Reduce model complexity, increase calculation speed, and compress some convolutional layers, which can further reduce model complexity, while ensuring the integrity of the model, and avoiding a sudden drop in model accurac...
PUM
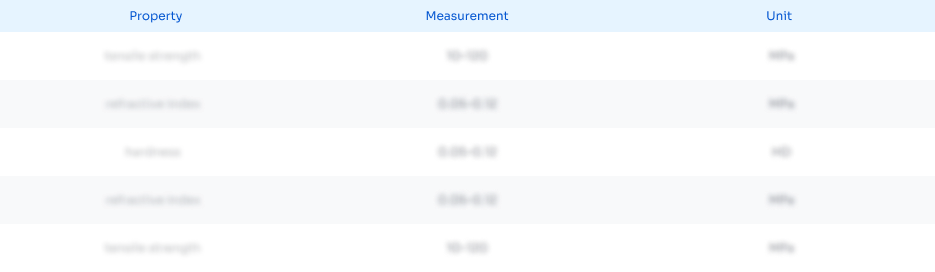
Abstract
Description
Claims
Application Information
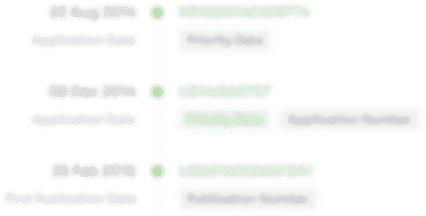
- Generate Ideas
- Intellectual Property
- Life Sciences
- Materials
- Tech Scout
- Unparalleled Data Quality
- Higher Quality Content
- 60% Fewer Hallucinations
Browse by: Latest US Patents, China's latest patents, Technical Efficacy Thesaurus, Application Domain, Technology Topic, Popular Technical Reports.
© 2025 PatSnap. All rights reserved.Legal|Privacy policy|Modern Slavery Act Transparency Statement|Sitemap|About US| Contact US: help@patsnap.com