Porosity prediction method based on selective ensemble learning
A technology that integrates learning and prediction methods, applied in the field of machine learning, to achieve the effect of easy implementation, easy implementation, and fast convergence speed
- Summary
- Abstract
- Description
- Claims
- Application Information
AI Technical Summary
Problems solved by technology
Method used
Image
Examples
Embodiment Construction
[0032] In order to make the object and technical solution of the present invention clearer, the implementation method of the present invention will be described in detail below in conjunction with the accompanying drawings.
[0033] The overall idea of the present invention is to propose a method of selective integrated learning to predict porosity for the problems of low error tolerance rate and overfitting in a single machine learning method for predicting porosity. In the above, a group of individual learning models with excellent performance are selected from classic models such as support vector regression, RBF neural network, random forest, ridge regression and K nearest neighbor regression through the "principal component method analysis" method to form an integrated learning model. The weights in the integrated learning model are obtained by the method of "principal component weight average", and finally the output of the integrated learning model is obtained by using...
PUM
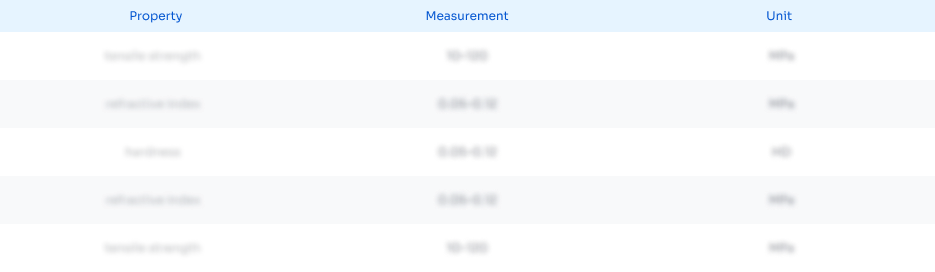
Abstract
Description
Claims
Application Information
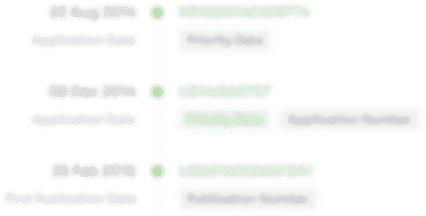
- R&D
- Intellectual Property
- Life Sciences
- Materials
- Tech Scout
- Unparalleled Data Quality
- Higher Quality Content
- 60% Fewer Hallucinations
Browse by: Latest US Patents, China's latest patents, Technical Efficacy Thesaurus, Application Domain, Technology Topic, Popular Technical Reports.
© 2025 PatSnap. All rights reserved.Legal|Privacy policy|Modern Slavery Act Transparency Statement|Sitemap|About US| Contact US: help@patsnap.com