Structural health monitoring data exception identification method based on space-time diagram convolutional network
A convolutional network, health monitoring technology, applied in neural learning methods, special data processing applications, biological neural network models, etc., can solve problems such as difficulty in distinguishing sensor faults and structural variation
- Summary
- Abstract
- Description
- Claims
- Application Information
AI Technical Summary
Problems solved by technology
Method used
Image
Examples
Embodiment 1
[0059] Such as figure 1 As shown, a method for identifying sensor faults and structural variations in a structural health monitoring system based on deep learning includes the following steps:
[0060] Step 1. Preprocess the cable force monitoring data of a cable-stayed bridge health monitoring system in the past four years, select and standardize the cable force trend item data of 42 consecutive cable force sensors, and take 5 minutes as the time interval, and consider 7 time step, create a dataset of training instances.
[0061] Step 2. Use the spatio-temporal graph convolutional network that can learn the adjacency matrix to model the spatio-temporal association of the structural monitoring data, use the information of different distance nodes for data regression in a hierarchical manner, and design the corresponding network structure and objective function penalty term;
[0062] Step 3. Use the measured data at the initial stage of the monitoring system (2006 to early 200...
PUM
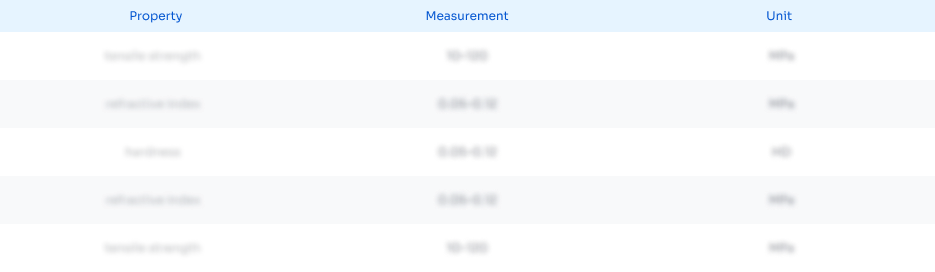
Abstract
Description
Claims
Application Information
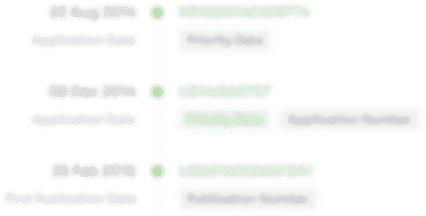
- R&D
- Intellectual Property
- Life Sciences
- Materials
- Tech Scout
- Unparalleled Data Quality
- Higher Quality Content
- 60% Fewer Hallucinations
Browse by: Latest US Patents, China's latest patents, Technical Efficacy Thesaurus, Application Domain, Technology Topic, Popular Technical Reports.
© 2025 PatSnap. All rights reserved.Legal|Privacy policy|Modern Slavery Act Transparency Statement|Sitemap|About US| Contact US: help@patsnap.com