Remote sensing image cloud detection method based on Gabor transformation and attention
A technology of remote sensing image and attention, which is applied in the field of image processing, can solve the problems of non-concentrated distribution of eigenvalues and complex types of ground objects, and achieve the effect of enhancing the ability of features, improving accuracy, and improving detection accuracy
- Summary
- Abstract
- Description
- Claims
- Application Information
AI Technical Summary
Problems solved by technology
Method used
Image
Examples
Embodiment 1
[0031] Satellite remote sensing technology has entered a new stage where it can quickly and timely obtain earth observation information, but the clouds in the image will block the ground objects, which will interfere with the ground object information obtained through satellite remote sensing images. In order to effectively To extract the information of ground target objects from remote sensing image data and improve the availability and utilization of remote sensing satellite image data, it is necessary to detect the clouds existing in remote sensing images, but because different types of clouds have different image feature values, plus There are many types of ground features, which have affected the accuracy of cloud detection. The present invention conducts research on these current situations, and proposes a deep learning remote sensing image cloud detection method based on Gabor transformation and attention mechanism, see figure 1 , figure 1 It is an implementation flow ...
Embodiment 2
[0053] The deep learning remote sensing cloud detection method based on Gabor transform and attention mechanism is the same as embodiment 1, step 2a) in constructing the Gabor transform module, the output feature map of the constructed Gabor transform branch passes through the output feature map of the convolution transform branch Perform a subtraction operation to obtain the information difference with the feature map of the convolution transformation branch, and re-add the information difference to the feature map in the convolution transformation branch after a convolution study to obtain the output of the Gabor transformation module . The kernel function of the Gabor filter is defined as follows:
[0054]
[0055]
[0056] In the formula, x and y represent the horizontal and vertical coordinates on the two-dimensional surface, λ represents the wavelength, θ represents the direction of the filter stripe, ψ represents the phase shift, σ represents the standard deviatio...
Embodiment 3
[0063] The deep learning remote sensing cloud detection method based on Gabor transformation and attention mechanism is the same as embodiment 1-2, step 2b) in constructing the attention mechanism module, the constructed attention mechanism module includes a space attention module and a channel attention module; space The attention module includes a convolutional layer, a channel splicing layer, a global maximum pooling layer, and a global average pooling layer; a global maximum pooling layer and a global average pooling layer are connected in parallel, and then a channel splicing layer and a volume are connected in series product layer; the channel attention module includes a convolutional layer, a global maximum pooling layer, a global average pooling layer, a fully connected layer, a pixel addition layer, and a Sigmoid function; a convolutional layer is connected in parallel with a global maximum pooling layer and A global average pooling layer, a global maximum pooling laye...
PUM
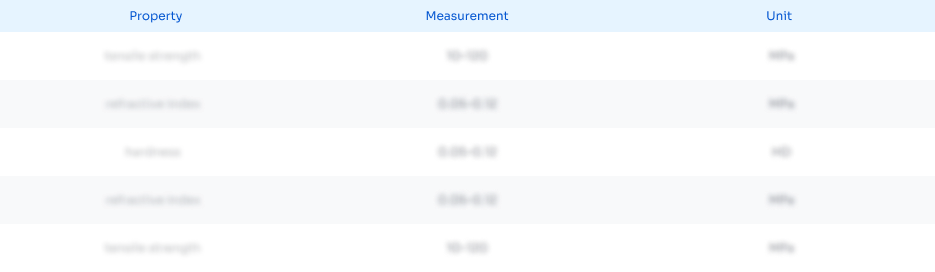
Abstract
Description
Claims
Application Information
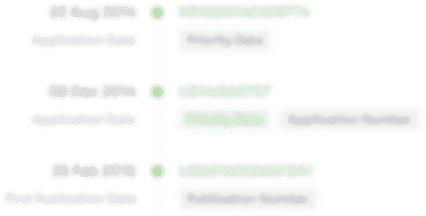
- R&D
- Intellectual Property
- Life Sciences
- Materials
- Tech Scout
- Unparalleled Data Quality
- Higher Quality Content
- 60% Fewer Hallucinations
Browse by: Latest US Patents, China's latest patents, Technical Efficacy Thesaurus, Application Domain, Technology Topic, Popular Technical Reports.
© 2025 PatSnap. All rights reserved.Legal|Privacy policy|Modern Slavery Act Transparency Statement|Sitemap|About US| Contact US: help@patsnap.com