Image super-resolution reconstruction method based on residual learning
A technology of super-resolution reconstruction and image reconstruction, which is applied in the field of deep learning, can solve the problems of reconstruction image quality, weak network learning ability, long training time, etc. The effect of increased structural accuracy
- Summary
- Abstract
- Description
- Claims
- Application Information
AI Technical Summary
Problems solved by technology
Method used
Image
Examples
Embodiment Construction
[0049] Below in conjunction with accompanying drawing and specific embodiment, further illustrate the present invention, should be understood that these examples are only for illustrating the present invention and are not intended to limit the scope of the present invention, after having read the present invention, those skilled in the art will understand various aspects of the present invention All modifications of the valence form fall within the scope defined by the appended claims of the present application.
[0050] An image super-resolution reconstruction method based on residual learning, such as figure 1 shown, including the following steps:
[0051] Step 1), obtain the public data set used for training the system. The data sets include DIV2K, Set5 and Set14, where DIV2K contains 800 training images, Set5 contains 100 verification images, and Set14 contains 100 test data sets, using 800 training data sets in DIV2K, Set5 and Set14 as test data sets, Each example data ...
PUM
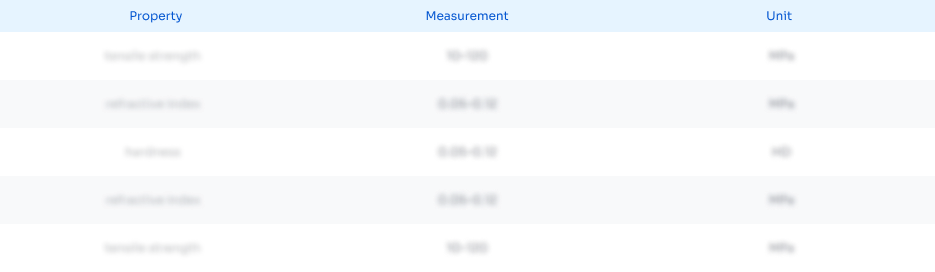
Abstract
Description
Claims
Application Information
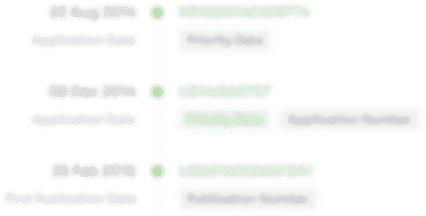
- R&D Engineer
- R&D Manager
- IP Professional
- Industry Leading Data Capabilities
- Powerful AI technology
- Patent DNA Extraction
Browse by: Latest US Patents, China's latest patents, Technical Efficacy Thesaurus, Application Domain, Technology Topic, Popular Technical Reports.
© 2024 PatSnap. All rights reserved.Legal|Privacy policy|Modern Slavery Act Transparency Statement|Sitemap|About US| Contact US: help@patsnap.com