Conveyor belt carrier roller detection method based on YOLOv3
A detection method and conveyor belt technology, applied in the field of computer vision and deep learning, can solve the problems of low detection accuracy of small targets, large training parameters, and large amount of calculation, so as to meet the real-time detection requirements, meet the accuracy requirements, The effect of improving detection accuracy
- Summary
- Abstract
- Description
- Claims
- Application Information
AI Technical Summary
Problems solved by technology
Method used
Image
Examples
Example Embodiment
[0052] Example 1
[0053] Such as figure 1 Shown is the overall flow chart of the training and testing of the present invention, which specifically includes the following steps:
[0054] S1. Before training, first prepare the data set required for training, collect belt idler pictures under different lighting, temperature and other environments to make an initial sample data set; specifically, step S1 includes:
[0055] S11. By installing cameras at different positions on both sides of the belt, real-time shooting of the picture information of the idler when the belt is rotating;
[0056] S12. Perform frame division processing on the acquired idler video, extract images of each frame, and obtain idler image sequence sets in different time periods;
[0057] S13. Filter the sequence set obtained in step S12, and select the roller image sequence set under different illumination, different time period, different weather, etc.; to obtain an initial sample data set.
[0058] S2. Perform prepro...
PUM
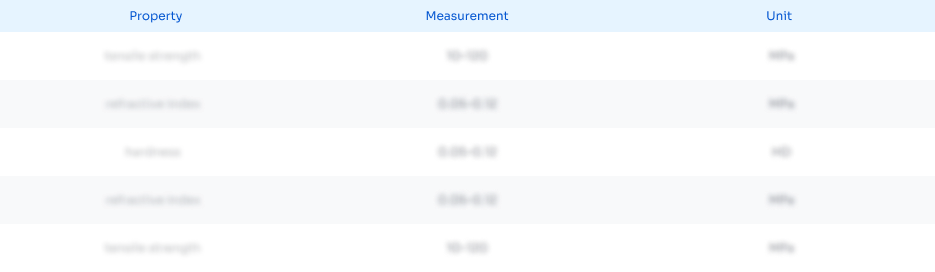
Abstract
Description
Claims
Application Information
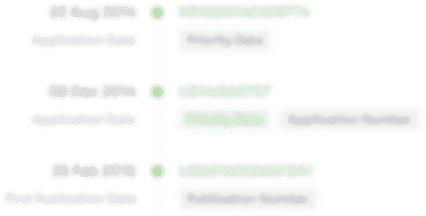
- R&D Engineer
- R&D Manager
- IP Professional
- Industry Leading Data Capabilities
- Powerful AI technology
- Patent DNA Extraction
Browse by: Latest US Patents, China's latest patents, Technical Efficacy Thesaurus, Application Domain, Technology Topic.
© 2024 PatSnap. All rights reserved.Legal|Privacy policy|Modern Slavery Act Transparency Statement|Sitemap