Chinese electronic medical record entity labeling method based on BIC
An electronic medical record and entity technology, applied in neural learning methods, electrical digital data processing, medical data mining, etc., can solve problems such as consuming a lot of manpower and material resources, and limited annotation corpus
- Summary
- Abstract
- Description
- Claims
- Application Information
AI Technical Summary
Problems solved by technology
Method used
Image
Examples
Embodiment 1
[0048] In this example, see figure 1 , a BIC-based Chinese electronic medical record entity labeling method, the specific steps are as follows:
[0049] 1) First give the corresponding medical entity labeling specifications according to actual needs, then manually label a small amount of data, and process the manually labeled data into the data format required by the model to form training data;
[0050] 2) Then train the model parameters and generate a sequence labeling model, which includes BiLSTM, Iterative Dilated Convolutional Neural Network (IDCNN), and Conditional Random Field (CRF), where BiLSTM and IDCNN are used as the encoding end of the model , CRF as the decoding end of the model;
[0051] 3) Input the data to be labeled into the sequence labeling model, output the result, and obtain the data labeled by the machine;
[0052] 4) Then manual review and correction of part of the labeling errors, and then through data processing operations, to obtain the training da...
Embodiment 2
[0055] This embodiment is basically the same as Implementation 1, the special features are:
[0056] In this example, see figure 1 , in the step 2), the method for generating the sequence labeling model is as follows:
[0057] a. The input of the model is Chinese text. According to different lengths of text, it is divided into different training batches. Each training batch has 20 sentences of text. A batch of training texts are converted into tensors through the embedding layer, and each batch of training texts is passed through Fill in the gap to achieve the same length;
[0058] b. The tensor of the input data obtained by the embedding layer is processed by the encoding end. The encoding end is formed by the combination of BiLSTM and IDCNN. The number of neurons in the hidden layer of BiLSTM is set, and the output of the BiLSTM layer corresponds to the tensor;
[0059] c. Input the output of the BiLSTM layer to the IDCNN layer to extract local detail features of the text;...
Embodiment 3
[0063] This embodiment is basically the same as Implementation 1, the special features are:
[0064] In this example, see figure 1 , a BIC-based Chinese electronic medical record entity labeling method, the process of the method is as follows figure 1 As shown, firstly, the corresponding medical entity labeling specifications are given according to the actual needs, and then a small amount of data is manually labeled, and the manually labeled data is processed into the data format required by the model to form training data. Then train the model parameters to generate a sequence labeling model. Input the data to be labeled into the sequence labeling model, output the results, and obtain the machine-labeled data, then manually review and correct some labeling errors, and then perform data processing operations to obtain the training data required for the model, and perform model training again. Since the deep learning model used becomes better and better with the increase of ...
PUM
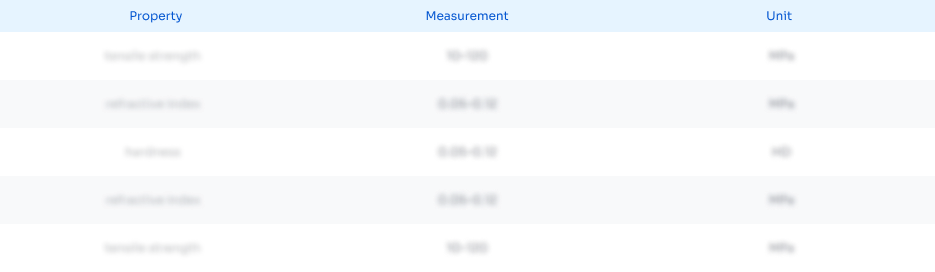
Abstract
Description
Claims
Application Information
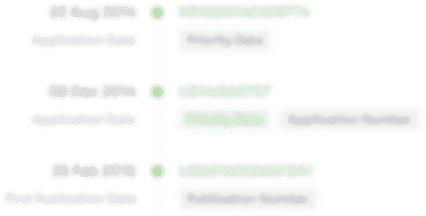
- R&D Engineer
- R&D Manager
- IP Professional
- Industry Leading Data Capabilities
- Powerful AI technology
- Patent DNA Extraction
Browse by: Latest US Patents, China's latest patents, Technical Efficacy Thesaurus, Application Domain, Technology Topic, Popular Technical Reports.
© 2024 PatSnap. All rights reserved.Legal|Privacy policy|Modern Slavery Act Transparency Statement|Sitemap|About US| Contact US: help@patsnap.com