Deep learning magnetic resonance spectrum reconstruction method based on sparse representation
A sparse representation, deep learning technology, applied in neural learning methods, magnetic resonance measurement, material analysis by resonance, etc., can solve the problem that the reconstruction quality needs to be improved, and the sparse characteristics of frequency domain signals have not yet been utilized.
- Summary
- Abstract
- Description
- Claims
- Application Information
AI Technical Summary
Problems solved by technology
Method used
Image
Examples
Embodiment Construction
[0048] The following embodiments will further illustrate the present invention in conjunction with the accompanying drawings. In the embodiment of the present invention, an exponential function is used to generate the spectrum corresponding to the fully sampled time domain signal as the training set label, the undersampled time domain signal and the corresponding undersampled template are used as the training set input, and the optimal network parameters are obtained through several iterations of training, Finally, the under-sampled data to be reconstructed is input into the network to obtain the reconstructed magnetic resonance spectrum.
[0049] Specific examples are given below.
[0050] Embodiments of the present invention include the following steps:
[0051] Step 1: Generating time-domain signals for magnetic resonance spectroscopy using exponential functions
[0052] In this embodiment, a total of 40,000 free induction attenuation signals are generated, and a fully sa...
PUM
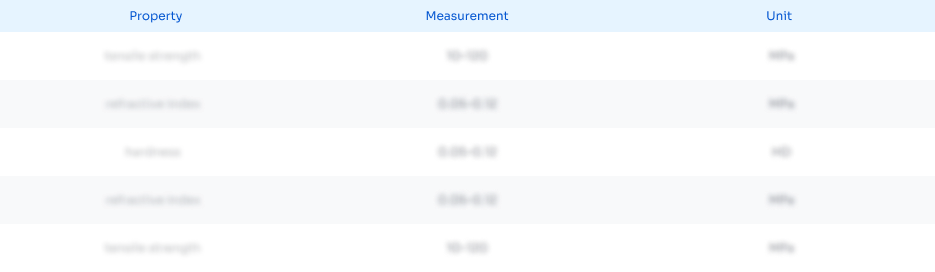
Abstract
Description
Claims
Application Information
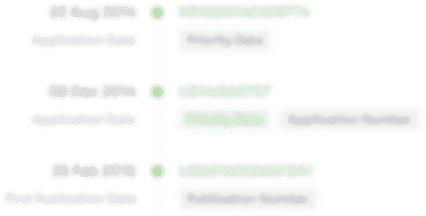
- R&D Engineer
- R&D Manager
- IP Professional
- Industry Leading Data Capabilities
- Powerful AI technology
- Patent DNA Extraction
Browse by: Latest US Patents, China's latest patents, Technical Efficacy Thesaurus, Application Domain, Technology Topic, Popular Technical Reports.
© 2024 PatSnap. All rights reserved.Legal|Privacy policy|Modern Slavery Act Transparency Statement|Sitemap|About US| Contact US: help@patsnap.com