Distracted driving recognition method based on federated learning
An identification method and federated technology, applied in the computer field, can solve problems such as poor communication between data owners and data analysts, failure to consider privacy protection issues, and users' reluctance to share, so as to reduce computing power dependence, solve data islands, The effect of meeting the needs of reality
- Summary
- Abstract
- Description
- Claims
- Application Information
AI Technical Summary
Problems solved by technology
Method used
Image
Examples
example 1
[0051] 1. Obtain open source datasets: Obtain relevant pictures and annotation information of distracted driving from public datasets such as ImageNet, Open Images, Image V5, such as image 3 shown.
[0052] Dataset setup mainly consists of three key steps:
[0053] (1) Collect image sets related to driver distraction behavior recognition;
[0054] (2) Preprocessing the images in the data set, such as rotation, translation, scaling, etc., to increase the diversity of data;
[0055] (3) Classify and label data graphics.
[0056] 2. Build the model: In the example, both the cloud server and the client execute DNN based on the TensorFlow Federated (TFF) framework. Meanwhile, for the client side, SCNN is implemented through TensorFlow.
[0057] 3. The specific time setting of the shallow mixed model is as follows: Figure 4 shown.
[0058] In order to verify the superiority of the present invention in distracted driving recognition, the algorithm proposed by the present inve...
PUM
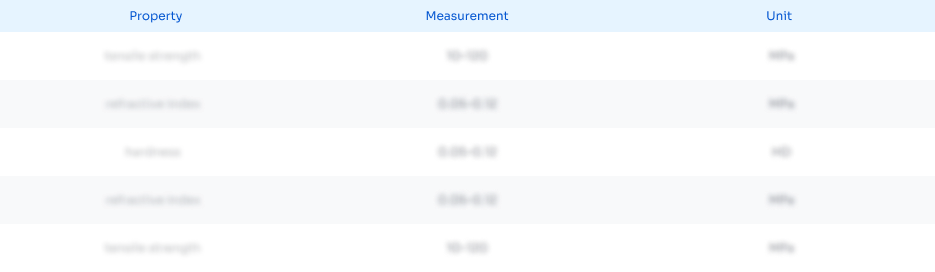
Abstract
Description
Claims
Application Information
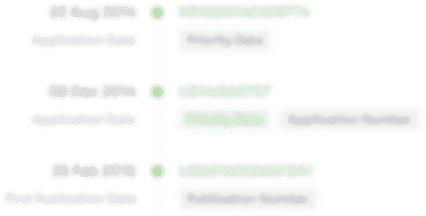
- R&D Engineer
- R&D Manager
- IP Professional
- Industry Leading Data Capabilities
- Powerful AI technology
- Patent DNA Extraction
Browse by: Latest US Patents, China's latest patents, Technical Efficacy Thesaurus, Application Domain, Technology Topic, Popular Technical Reports.
© 2024 PatSnap. All rights reserved.Legal|Privacy policy|Modern Slavery Act Transparency Statement|Sitemap|About US| Contact US: help@patsnap.com