Magnetic resonance imaging method, device and system and storage medium
A magnetic resonance imaging and magnetic resonance image technology, applied in the field of deep learning, can solve the problems of limited parallel imaging acceleration multiple, image noise amplification, difficult selection of sparse transformation and reconstruction parameters, etc., to achieve the effect of improving the degree of freedom and quality
- Summary
- Abstract
- Description
- Claims
- Application Information
AI Technical Summary
Problems solved by technology
Method used
Image
Examples
Embodiment 1
[0030] figure 2 It is a schematic flowchart of a magnetic resonance imaging method provided in Embodiment 1 of the present invention. This embodiment is applicable to the case of magnetic resonance imaging based on a neural network, and the method can be performed by the magnetic resonance imaging device provided in the embodiment of the present application. Specifically include the following steps:
[0031] S110. Acquire an original model of magnetic resonance imaging, and establish an initial imaging model according to an iterative algorithm for solving the original model, where the iterative algorithm includes at least one of undetermined parameters, undetermined solution operators, and undetermined structural relationships.
[0032] S120. Train the initial imaging model based on sample data to generate a magnetic resonance imaging model, wherein the training of the initial imaging model is used to learn undetermined parameters, undetermined solution operators and undeterm...
Embodiment 2
[0067] Figure 9 It is a schematic structural diagram of a magnetic resonance imaging device provided in Embodiment 2 of the present invention, and the magnetic resonance imaging device includes:
[0068] The initial imaging model building module 210 is used to obtain the original model of the magnetic resonance imaging, and establish the initial imaging model according to the iterative algorithm for solving the original model, the iterative algorithm includes undetermined parameters, undetermined solution operators and undetermined structural relationships at least one of;
[0069] A model training module 220, configured to train the initial imaging model based on sample data to generate a magnetic resonance imaging model, wherein the training of the initial imaging model is used to learn undetermined parameters and undetermined solution operators in the iterative algorithm and at least one of the pending structural relationships;
[0070] The magnetic resonance imaging mod...
Embodiment 3
[0091] Figure 10 It is a schematic structural diagram of a magnetic resonance system provided in Embodiment 3 of the present invention, Figure 10 A block diagram of an exemplary medical imaging system suitable for implementing embodiments of the invention is shown, Figure 10 The medical imaging system shown is only an example, and should not impose any limitation on the functions and scope of use of the embodiments of the present invention.
[0092] The magnetic resonance system includes a magnetic resonance device 300 and a computer 400 .
[0093] The computer 400 can be used to implement specific methods and devices disclosed in some embodiments of the present invention. The specific device in this embodiment uses a functional block diagram to show a hardware platform including a display module. In some embodiments, the computer 400 can implement some embodiments of the present invention through its hardware devices, software programs, firmware and their combinations. ...
PUM
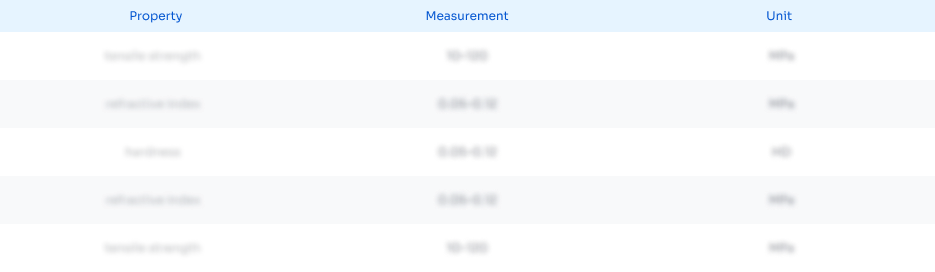
Abstract
Description
Claims
Application Information
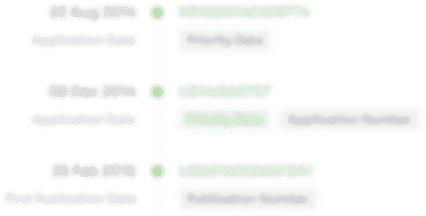
- R&D
- Intellectual Property
- Life Sciences
- Materials
- Tech Scout
- Unparalleled Data Quality
- Higher Quality Content
- 60% Fewer Hallucinations
Browse by: Latest US Patents, China's latest patents, Technical Efficacy Thesaurus, Application Domain, Technology Topic, Popular Technical Reports.
© 2025 PatSnap. All rights reserved.Legal|Privacy policy|Modern Slavery Act Transparency Statement|Sitemap|About US| Contact US: help@patsnap.com