Generative adversarial network model and vehicle trajectory prediction method using same
A network model and vehicle trajectory technology, which is applied to and utilizes the vehicle trajectory prediction of the generated confrontation network model, and the field of generating the confrontation network model, which can solve the problems of low precision, few input features, and low robustness, so as to improve the accuracy Sexuality, strengthening constraints, and avoiding the effect of overfitting
- Summary
- Abstract
- Description
- Claims
- Application Information
AI Technical Summary
Problems solved by technology
Method used
Image
Examples
Embodiment Construction
[0018] The present invention will be further described below in conjunction with accompanying drawing.
[0019] The present invention proposes a vehicle trajectory prediction method based on a generative confrontation network, and the process of realizing the method is as follows figure 2 shown, including the following steps:
[0020] S1: Design a generative confrontation network model;
[0021] S2: Generate processing data and input the data into the generated confrontation network model.
[0022] S3: Use the input data to train the generated confrontation network model.
[0023] S4: Use the verification data to verify the GAN model trained in step S2. If the verification result is satisfactory, this network model can be used for vehicle trajectory prediction. Otherwise, continue to execute S2 until the verification result is satisfactory.
[0024] Embodiments will be specifically described below.
[0025] The design of step S1 generates an adversarial network model su...
PUM
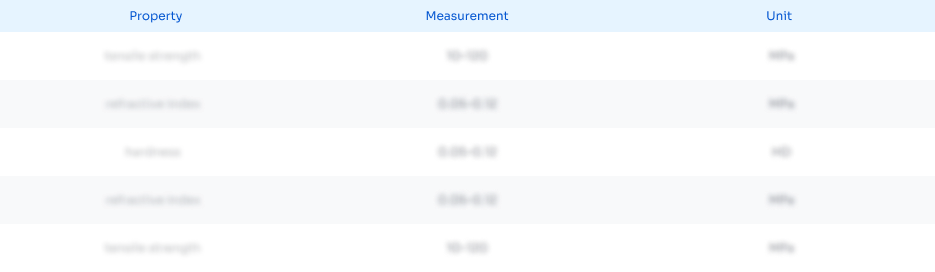
Abstract
Description
Claims
Application Information
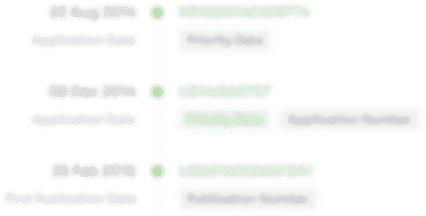
- R&D Engineer
- R&D Manager
- IP Professional
- Industry Leading Data Capabilities
- Powerful AI technology
- Patent DNA Extraction
Browse by: Latest US Patents, China's latest patents, Technical Efficacy Thesaurus, Application Domain, Technology Topic, Popular Technical Reports.
© 2024 PatSnap. All rights reserved.Legal|Privacy policy|Modern Slavery Act Transparency Statement|Sitemap|About US| Contact US: help@patsnap.com