Fine-grained image retrieval method based on self-attention mechanism weighting
An image retrieval and attention technology, which is applied in the fields of image retrieval and computer vision, can solve the problems of high computational time consumption and inaccurate selection of target features in the visual attention method, and achieve the effect of improving retrieval accuracy and reducing computational complexity
- Summary
- Abstract
- Description
- Claims
- Application Information
AI Technical Summary
Problems solved by technology
Method used
Image
Examples
Embodiment Construction
[0023] 1. Image preprocessing
[0024] The present invention does not have specific requirements on the resolution of the image, but for consideration of retrieval efficiency, the longest side length of the image is set as an upper limit of 500 pixels during implementation. When the image needs to be scaled down, the original aspect ratio will be maintained. In addition, use the data provided by the ImageNet data to perform zero-mean processing on the pixel values on each channel of the image.
[0025] 2. Obtain the output of the convolutional neural network
[0026] The present invention is an unsupervised method and therefore only uses convolutional neural networks pre-trained in the ImageNet dataset. After inputting the image into the network, the output of the last convolutional layer is selected, and a three-dimensional tensor T of shape h×w×c can be obtained. The 3D tensor output by a convolutional network has two widely used concepts: (1) a feature map consisting o...
PUM
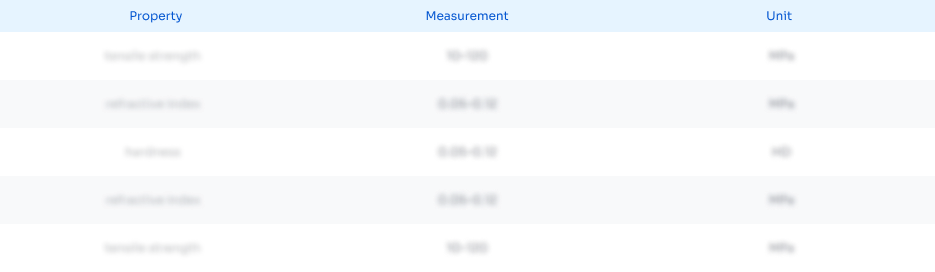
Abstract
Description
Claims
Application Information
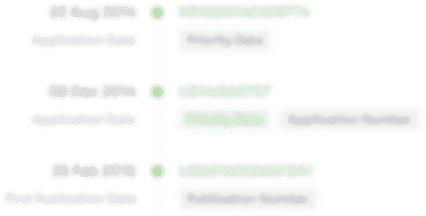
- Generate Ideas
- Intellectual Property
- Life Sciences
- Materials
- Tech Scout
- Unparalleled Data Quality
- Higher Quality Content
- 60% Fewer Hallucinations
Browse by: Latest US Patents, China's latest patents, Technical Efficacy Thesaurus, Application Domain, Technology Topic, Popular Technical Reports.
© 2025 PatSnap. All rights reserved.Legal|Privacy policy|Modern Slavery Act Transparency Statement|Sitemap|About US| Contact US: help@patsnap.com