Attribute graph group discovery method based on maximized mutual information and graph neural network
A technology that maximizes mutual information and neural networks, applied in the field of big data, can solve problems such as unsupervised difficulties and lack of modeling, achieve high accuracy and facilitate iterative updates
- Summary
- Abstract
- Description
- Claims
- Application Information
AI Technical Summary
Problems solved by technology
Method used
Image
Examples
Embodiment Construction
[0031] In order to make the technical means, creative features, goals and effects achieved by the present invention easy to understand, the method for discovering attribute graph groups based on maximizing mutual information and graph neural networks of the present invention will be described in detail below in conjunction with the embodiments and accompanying drawings.
[0032]
[0033] figure 1 It is a flow chart of an attribute graph group discovery method based on maximizing mutual information and a graph neural network according to an embodiment of the present invention.
[0034] Such as figure 1 As shown, the process of the attribute graph group discovery method based on maximizing mutual information and graph neural network includes the following steps:
[0035]Step S1, obtaining node information of each node in the attribute graph to be processed, and processing all node information to obtain a matrix to be processed including an adjacency matrix and an attribute ma...
PUM
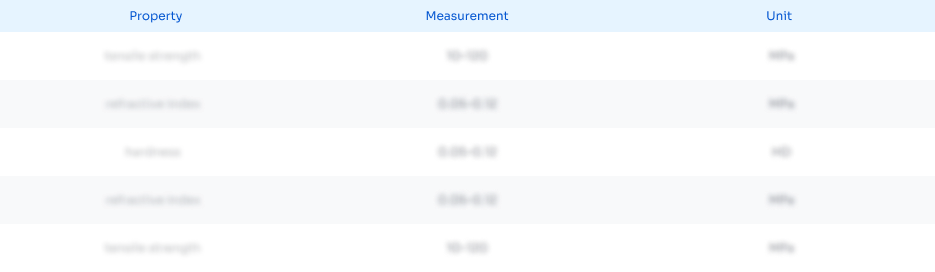
Abstract
Description
Claims
Application Information
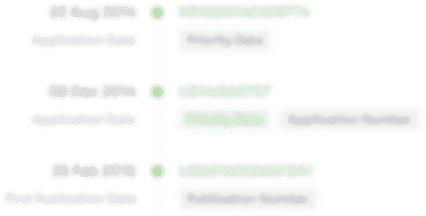
- R&D Engineer
- R&D Manager
- IP Professional
- Industry Leading Data Capabilities
- Powerful AI technology
- Patent DNA Extraction
Browse by: Latest US Patents, China's latest patents, Technical Efficacy Thesaurus, Application Domain, Technology Topic.
© 2024 PatSnap. All rights reserved.Legal|Privacy policy|Modern Slavery Act Transparency Statement|Sitemap