Pine nut protein content prediction method based on ensemble learning calibration model
A technique for protein content, calibration models
- Summary
- Abstract
- Description
- Claims
- Application Information
AI Technical Summary
Problems solved by technology
Method used
Image
Examples
specific Embodiment approach 1
[0024] Specific implementation mode one: combine Figure 5 This embodiment will be described. A method for predicting protein content of pine nuts based on an integrated learning calibration model described in this embodiment, the method is specifically implemented through the following steps:
[0025] Step 1, collect the original spectral data samples of the pine nut sample, the number of samples is m, and then preprocess each original spectral data sample respectively, and obtain the preprocessed near-infrared spectral data;
[0026] Step 2. Use LTSA (Local Tangent Space Alignment), isomap (Isometric Feature Mapping), LLE (Local Linear Embedding) and PCA (Principal Component Analysis) to extract the features of the preprocessed near-infrared spectral data, and obtain four The four sets of feature vectors extracted by the method;
[0027] Step 3, select the boosting integrated learning algorithm to establish a calibration model for pine nut protein content prediction, and t...
specific Embodiment approach 2
[0031] Embodiment 2: This embodiment is a further detailed description of Embodiment 1. In the first step, each original spectral data sample is preprocessed respectively, and the method used in the preprocessing is standard normal variate , SNV) and SG (Savitzky-Golay) smoothing filtering.
[0032] In this embodiment, the near-infrared spectral data of the pine nut sample is preprocessed, the purpose of which is to eliminate the interference of sample surface scattering, baseline drift and noise on the spectral data, and enhance the data difference. Obtain near-infrared spectral data after removing interference.
specific Embodiment approach 3
[0033] Specific implementation mode three: this implementation mode is a further detailed description of specific implementation mode one, and the specific process of the step one is:
[0034] Step one, figure 2 Be the original spectrum of the pine nut sample collected by the spectrometer, for the i-th original spectrum data sample, set the optical program position number as j, j=1,2,...,l, l represents the total number of optical paths, Near-infrared spectral data obtained by fitting the original spectral data at the jth optical path position by using a p-order polynomial;
[0035]
[0036] Among them, a j′ is the weight coefficient, j'=0,1,...,p, the window width of p-order polynomial fitting is 2q+1, and λ is the absorbance in the wavelength range of the window width;
[0037] When the value of q is 4, the window width is 9, and when fitting the original spectral data at the 5th optical path position, then λ is the absorbance within the wavelength range from the 1st ...
PUM
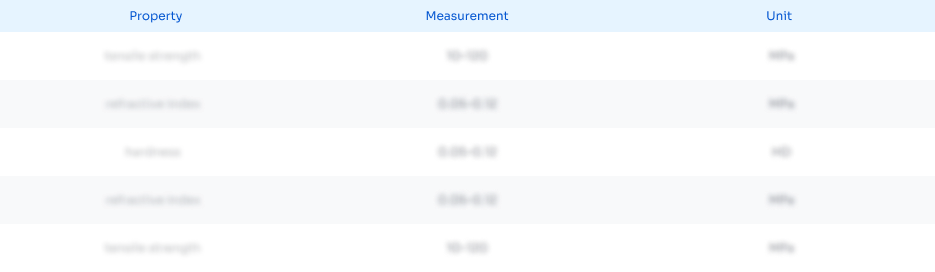
Abstract
Description
Claims
Application Information
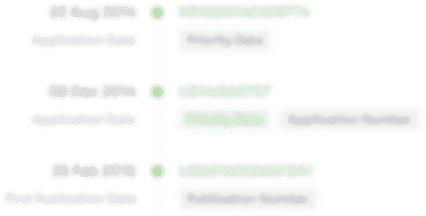
- R&D Engineer
- R&D Manager
- IP Professional
- Industry Leading Data Capabilities
- Powerful AI technology
- Patent DNA Extraction
Browse by: Latest US Patents, China's latest patents, Technical Efficacy Thesaurus, Application Domain, Technology Topic, Popular Technical Reports.
© 2024 PatSnap. All rights reserved.Legal|Privacy policy|Modern Slavery Act Transparency Statement|Sitemap|About US| Contact US: help@patsnap.com