Tool state image classification method based on edge mark graph neural network
A neural network and classification method technology, applied in the field of tool state image classification, can solve problems such as difficulties, non-stationary tool signals, and inability to make full use of state information
- Summary
- Abstract
- Description
- Claims
- Application Information
AI Technical Summary
Problems solved by technology
Method used
Image
Examples
Embodiment Construction
[0039] In order to make the object, technical solution and advantages of the present invention clearer, the present invention will be further described in detail below in conjunction with the accompanying drawings.
[0040] Such as figure 1 As shown, in the embodiment of the present invention, the following steps are included:
[0041] (1) Arrange and adjust the lighting system, set the magnification ratio of the lens, adjust the position of the tool to be tested, and then use a high-definition electronic measurement microscope to capture the tool wear image when the machine tool is shut down. The tool wear diagram is shown below:
[0042] (2) All the tool wear images captured in the experiment are divided into initial wear, normal wear, and sharp wear according to the size of the wear area.
[0043] (3) The pixels of the original image are large and there are many useless backgrounds, and preprocessing such as image cropping is performed. Then perform data normalization an...
PUM
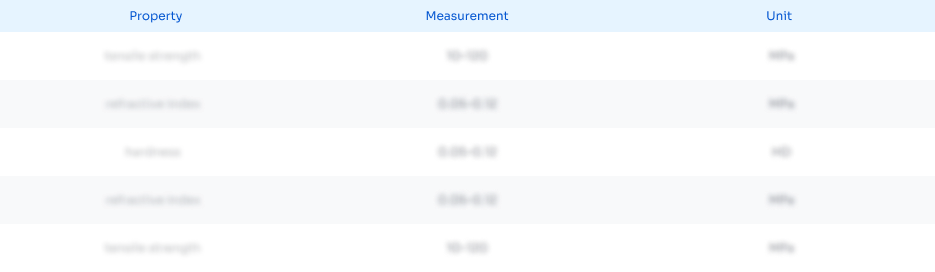
Abstract
Description
Claims
Application Information
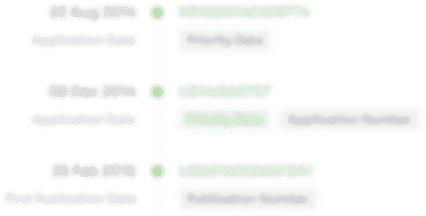
- R&D Engineer
- R&D Manager
- IP Professional
- Industry Leading Data Capabilities
- Powerful AI technology
- Patent DNA Extraction
Browse by: Latest US Patents, China's latest patents, Technical Efficacy Thesaurus, Application Domain, Technology Topic, Popular Technical Reports.
© 2024 PatSnap. All rights reserved.Legal|Privacy policy|Modern Slavery Act Transparency Statement|Sitemap|About US| Contact US: help@patsnap.com